DeepMind AI solves 50-year-old biology problem in breakthrough advance
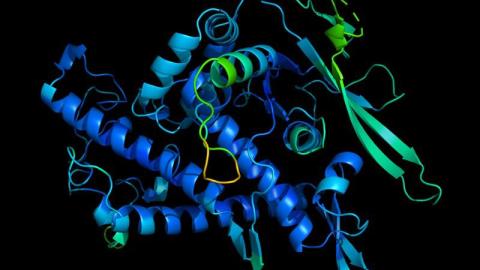
Credit: DeepMind
- Scientists have long been puzzled by how specific chains of amino acids go on to form three-dimensional proteins.
- DeepMind developed a system that’s able to predict “protein folding” in a fraction of the time of human experiments, and with unprecedented accuracy.
- The achievement could greatly improve drug research and development, as well as bioengineering pursuits.
In 1994, a group of scientists created a competition to solve one of the most perplexing problems in biology: how do proteins fold themselves into 3D shapes, which then carry out fundamental processes within living organisms?
The answer to this 50-year-old question could revolutionize many scientific pursuits, from accelerating and improving drug development, to creating better biofuels. But the competition, called Critical Assessment of Protein Structure Prediction (CASP), went decades without a solution.
Then artificial intelligence got into the mix.
DeepMind, a U.K.-based AI company, essentially solved the long-standing problem in the most recent competition, CASP14. The company outperformed the other teams by magnitudes, predicting the shapes of proteins with accuracy rates never before achieved by humans.
“This is a big deal,” John Moult, a computational biologist who co-founded CASP, told Nature. “In some sense the problem is solved.”
Credit: DeepMind
In the biennial competition, teams analyze around 100 proteins with the goal of predicting their eventual 3D shape. A protein’s shape determines its function. For example, a protein can become an antibody that binds to foreign particles to protect, an enzyme that carries out chemical reactions, or a structural component that supports cells.
Proteins start as a string of hundreds of amino acids. Within a protein, pairs of amino acids can interact in numerous ways, and these particular interactions determine the final shape of the protein. But given the sheer number of possible interactions, it’s incredibly difficult to predict a protein’s physical shape. Difficult, but not impossible.
Since CASP began, scientists have been able to predict the shape of some simple proteins with reasonable accuracy. CASP is able to verify the accuracy of these predictions by comparing them to the actual shape of proteins, which it obtains through the unpublished results of lab experiments.
But these experiments are difficult, often taking months or years of hard work. The shapes of some proteins have eluded scientists for decades. As such, it’s hard to overstate the value of having an AI that’s able to churn out this work in just hours, or even minutes.
In 2018, DeepMind, which was acquired by Google in 2014, startled the scientific community when its AlphaFold algorithm won the CASP13 contest. AlphaFold was able to predict protein shapes by “training” itself on vast amounts of data on known amino acid strings and their corresponding protein shapes.
In other words, AlphaFold learned that particular amino acid configurations—say, distances between pairs, angles between chemical bonds—signaled that the protein would likely take a particular shape. AlphaFold then used these insights to predict the shapes of unmapped proteins. AlphaFold’s performance in the 2018 contest was impressive, but not reliable enough to consider the problem of “protein folding” solved.
Credit: DeepMind
In the latest contest, DeepMind used an updated version of AlphaFold. It combines the previous deep-learning strategy with a new “attention algorithm” that accounts for physical and geometric factors. Here’s how DeepMind describes it:
“A folded protein can be thought of as a ‘spatial graph,’ where residues are the nodes and edges connect the residues in close proximity. This graph is important for understanding the physical interactions within proteins, as well as their evolutionary history.”
“For the latest version of AlphaFold, used at CASP14, we created an attention-based neural network system, trained end-to-end, that attempts to interpret the structure of this graph, while reasoning over the implicit graph that it’s building. It uses evolutionarily related sequences, multiple sequence alignment (MSA), and a representation of amino acid residue pairs to refine this graph.”
CASP measures prediction accuracy through the “Global Distance Test (GDT)”, which ranges from 0-100. The new version of AlphaFold scored a median of 92.4 GDT for all targets.
AlphaFold: The making of a scientific breakthroughyoutu.be
Given that the specific ways in which proteins take shape can shed light on how diseases form, AlphaFold could greatly accelerate disease research and drug development. And while it’s too late for the system to help with COVID-19, DeepMind says that protein structure prediction could be “useful in future pandemic response efforts.”
Still, scientists have much to learn about predicting protein structures, and while AlphaFold has proven faster and more accurate than human experiments, the system isn’t 100 percent accurate. But DeepMind’s achievement signals that AI may become a surprisingly powerful tool in unlocking key mysteries in biology and beyond.
“For all of us working on computational and machine learning methods in science, systems like AlphaFold demonstrate the stunning potential for AI as a tool to aid fundamental discovery,” DeepMind wrote. “Just as 50 years ago Anfinsen laid out a challenge far beyond science’s reach at the time, there are many aspects of our universe that remain unknown. The progress announced today gives us further confidence that AI will become one of humanity’s most useful tools in expanding the frontiers of scientific knowledge, and we’re looking forward to the many years of hard work and discovery ahead!”