Contact-tracing apps have serious physical, biological limitations
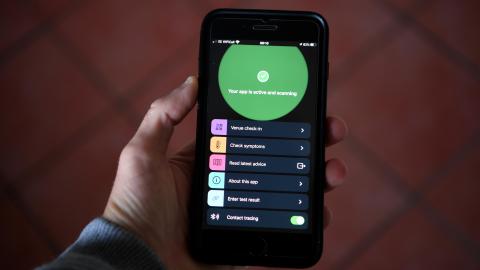
Credit: Gareth Copley via Getty Images
- The COVID-19 pandemic witnessed the widespread adoption of contact-tracing apps.
- Research shows that these apps aren’t as accurate as we might think.
- There are several physical and biological factors than can interfere with the accuracy of contact-tracing apps.
The following is an excerpt adapted from People Count: Contact-Tracing Apps and Public Health.
To stop an epidemic, public health authorities focus on lowering R0. Even for a given illness, this number varies tremendously according to the protective measures a society takes (wearing masks, practicing social distancing, and other measures). In early March 2020, COVID-19’s R0 was just below 4 in New York State. Once the state instituted a shelter-in-place order and virtually no one was on the streets, R0 dropped below 1. It continued to hover quite close to 1 for the summer and into the fall, even after the state came back to life and began to open bars and restaurants.
By helping to keep spread in check, could contact-tracing apps have lowered R0 enough to allow people to safely work, participate in social life, and be with their families? Lacking a real-life human experiment to answer this question, epidemiologists turn to models; these are in turn based on existing data. In the case of COVID-19, some of the best data comes from a citizen-science app developed by the BBC to accompany its 2018 documentary on the Spanish flu, Contagion! The BBC4 Pandemic. Participants agreed to provide a twenty-four-hour snapshot of their locations and self-reported contacts, which epidemiologists then used to model how a similar epidemic would spread in twenty-first-century Britain.
The BBC database ultimately included the locations and contacts of 36,000 people. It showed their movements over the course of a day, including how many people they saw at work, at school, and elsewhere. The data allowed researchers to develop a model that could simulate various interventions at the population level, from isolation, testing, contact tracing, and social distancing to app usage.
The resulting model showed that if 90 percent of ill people self-isolated and their household quarantined upon learning of their infection, 35 percent of cases would have already spread the disease to another person. If 90 percent of the contacts of those infected also isolated upon learning of the previous person’s infection, only 26 percent of cases would have infected someone else. The contact tracers, in other words, bought time. By having potentially infected people isolate, contact tracing prevented new rounds of infections. In another iteration, the researchers added apps to the mix and assumed that 53 percent of the population would use them. By notifying people of potential infections faster than a contact tracer could, the apps lowered the infection rate further, so that only 23 percent of cases infected another person. At that high adoption rate, the disease doesn’t disappear, but it also doesn’t cause a pandemic.
Models, of course, are only as good as the assumptions on which they’re based. The idea that 53 percent of any given population would voluntarily use a contact-tracing app and that anyone receiving an exposure notification would isolate is doubtful, at best. Still, because the apps appear to help lower R0, governments and public health officials have jumped to add them to the mix of public health tools available to combat COVID-19’s spread.
Signal strength varied depending on whether a person carried their phone in their back pocket, their front pocket, or in a backpack or handbag. The signal strength varied by device model, by the shape of the room, even by the construction materials.
Given the high stakes involved, we need to look at how apps are deployed in real life. How well do apps actually work? Are they more effective than more traditional, and less invasive, public health tools? Can they usefully supplement manual contact-tracing efforts? COVID-19 has hit low-income and Black, Latinx, and indigenous communities particularly hard. The possibility of public health organizations embracing contact-tracing apps as a line of defense against epidemics raises new questions about equity and the balance of individual privacy and public safety. Will contact-tracing apps exacerbate inequities already present in society?
A robust public debate about the implications of deploying what is effectively a public surveillance system didn’t occur; instead, many officials deployed these apps essentially overnight. We need that debate, but first we must look at efficacy. If the apps aren’t efficacious, then there is no reason to consider them further.
The many problems with contact-tracing apps
Following advice from the WHO, most public health agencies have promoted the idea that “social distancing” is the safest way to guard against exposure to the coronavirus. For the CDC, the magic number is six feet (in metric-based nations, it’s usually two meters). Stay at least that far away from other people, so the theory goes, and you’re safe. Since the BLE [Bluetooth Low Energy] technology on which contact-tracing apps run depends on proximity, engineers hoped that phone-to-phone contacts could serve as a reasonable proxy for risky exposures. In practice, this has turned out to be not entirely straightforward.
In theory, the strength of the BLE signal that a phone receives from another indicates the distance of the device emitting it. To test the accuracy of this assumption, researchers at Germany’s Fraunhofer-Gesellschaft simulated the experiences of people sitting on a train, waiting on line, being served by a waiter in a restaurant, and attending a cocktail party. Over 139 tests, the phones correctly determined time and distance exposure 70 percent of the time. This information seems encouraging, but the simulation took place in a test facility that lacked walls. The “train car” had no metal sides, the people waiting on line encountered no checkout counters or supermarket shelves, and neither the restaurant nor the cocktail party included walls or serving stations. This matters because radio waves often reflect off surfaces.
When researchers from the University of Dublin tried these tests in actual train cars, they obtained different results. Seven volunteers with phones running GAEN [(Google/Apple) Exposure Notification]-based apps distributed themselves around a train car and measured the signals their phones received over a fifteen-minute period. Radio waves are supposed to vary inversely according to the square of distance, so the researchers were surprised to find that the signals stayed constant at a distance of 1.5–2.5 meters and began to increase after that. Apparently, a flexible metal joint between train carriages concentrated the signal.
As they looked more closely at the results, the researchers found more surprises. Signal strength varied depending on whether a person carried their phone in their back pocket, their front pocket, or in a backpack or handbag. The signal strength varied by device model, by the shape of the room, even by the construction materials. Depending on the construction material, BLE signals can indicate that people are near each other when they are actually in neighboring apartments.
Epidemiologists understand that the six-foot measure is somewhat arbitrary; engineers know that BLE signals don’t measure distances precisely. If the rest of us come to use these systems, we also need to understand their limitations.
Apps don’t account for real-life circumstances
Measurement imprecision isn’t the only problem for contact-tracing and exposure-notification apps. The apps are not built to record the real-life circumstances that affect the likelihood of transmission in any given case. If Alyssa is six feet away from Ben in a small room for fifteen minutes, there’s likely risk of exposure. But if Alyssa is four feet from Ben, outside, and wearing a mask, she’s likely to be safe. Large gatherings of people indoors carry risks of spread, while similarly sized groups of masked people outdoors are less dangerous. Apps can’t distinguish between these situations. Nor do apps know if the person standing eight feet away from you is belting out a song — dangerous if they’re infected — or just standing quietly.
The apps are also ignorant of a room’s ventilation, an important factor in how the virus spreads. When an infected person breathes — or speaks, sings, coughs, or sneezes — they emit viral particles packaged in a mixture of mucus, saliva, and water. The smallest of these, aerosols, evaporate as they travel, losing some of their potency. The bigger ones, droplets, typically fall to the ground within three feet. Sometimes, though, air flow, particularly air conditioning, can push these along, putting people at further distances at risk of infection. This is apparently what happened in a restaurant in Guangzhou, China, when two people sitting well beyond the six-foot measure — and on different sides of the ill person — were infected. One was at a table more than a dozen feet away.
Biology also confuses apps. A review of published reports indicates that as many as 30–40 percent of people never show symptoms. While these studies are not based on random samples, a single study based on a large random sample of Icelanders showed a similar result: a startling 43 percent of participants tested positive without showing symptoms. Even if one assumes that only 30 percent of cases are asymptomatic — a not unreasonable assumption — then epidemiologists believe that 7 percent of transmission will arise from asymptomatic cases. This matters for the apps’ effectiveness. Asymptomatic people are less likely to get tested than those who are sick — and if there’s no test, there’s no trigger for exposure notifications.
Contact-tracing and exposure-notification apps nevertheless do have value. They pick up cases that people, including contact tracers, wouldn’t. Aliyah might not remember a chance hallway encounter with Bobby, but her app will. And the app will be ready to notify Aliyah if Bobby’s phone reports a positive COVID-19 test. Perhaps even more critically, Aliyah’s app will register encounters with nearby strangers in the bar or theater lobby — as long as they are also using the app. If those strangers later test positive, Aliyah will learn she’s been exposed. Without a phone app, she’d have little chance of discovering this.
False positives and false negatives
These technical and practical limitations of contact-tracing apps mean that they can produce both false positives and false negatives. (Note that these are false positives and false negatives of exposure, not false positive and false negatives of having COVID-19.) Virginia’s website for the state’s GAEN-based app, for example, warns that students in adjacent dorm rooms might receive exposure notifications of close contact while being in different rooms. When tested in August 2020, the UK exposure-notification app had a 45 percent false positive rate and 31 percent false negative rate.
These numbers sound bad, but the false positives aren’t entirely “false” — most of them represented exposures at 2.5–4 meters away rather than 2 meters. Depending on the circumstances, a person might well have been exposed at 3 meters. In the case of false negatives, however, users received no notification whatsoever that they had been in the presence of someone infected with COVID-19.
The apps are not built to record the real-life circumstances that affect the likelihood of transmission in any given case.
Both types of inaccuracies present challenges for users and public health agencies — some more obvious than others. If Aliyah receives a false positive notification, she might quarantine unnecessarily, losing a paycheck. If she’s following the rules, she should also urge her roommates and family members she’s in close contact with to do so, causing more disruption. Alternatively, if this is the second time that the app warns Aliyah that she’s been exposed without her developing any symptoms, she might just ignore the notification and disable the app.
False negatives place the public’s health at risk. If Bobby was asymptomatic and never tested, Aliyah will not receive a notification even though she may have spent fifty minutes sitting six feet away from Bobby in a classroom. False negatives can also be produced by circumstance: from an air conditioner dispersing aerosols farther than expected or an infected singer who propels droplets farther than six feet.
Some communities are at higher risk for false positives than others. Many low-income people, for instance, hold jobs that bring them in constant contact with a stream of strangers (e.g., grocery store clerks, health care workers, workers in food service and production). For these workers, a small variation in the proximity measurement (say, nine feet instead of six) can multiply into a high risk of false positives from contact-tracing apps. What’s more, many of these workers routinely wear protective gear or work behind barriers that reduce their risk from even four-foot interactions. Similarly, people who live in high-density housing situations, whether multifamily housing units or apartment complexes, are more likely to receive false positives than people who live in stand-alone suburban or rural houses.
Hourly workers living paycheck to paycheck can’t afford to take time off unless it’s absolutely necessary. A false positive keeps them from clocking in. Alyssa, in Singapore, or Amelie, in Switzerland, can each expect to receive financial support from the government if they isolate after an exposure notification. But in the United States, few low-income or gig workers receive paid time off, even for isolating during a pandemic. The privilege of staying at home is not evenly distributed. Workers who realize that the apps consistently generate false positives are less likely to use them voluntarily — or to heed them when they provide alerts.
False negatives, too, have a differential impact. White-collar workers who already work from home and who drive their own vehicles on necessary errands have fewer contacts than those who take public transportation to jobs that have been deemed “essential.” The fewer contacts each of us has with other people, the less chance we have of spreading COVID-19. A false negative of exposure for someone who works outside the home and uses public transit carries greater risk of infecting others than the same false negative for someone who works at home and uses their own transportation.
Contact-tracing apps were supposed to resolve this problem, allowing people to emerge from lockdowns with the ability to interact with friends, family, and strangers. It’s not clear that they will.
Adapted excerpt from People Count: Contact-Tracing Apps and Public Health by Susan Landau. Reprinted with Permission from The MIT PRESS. Copyright 2021.