How do “genes” work? So-called experts have a hard time agreeing
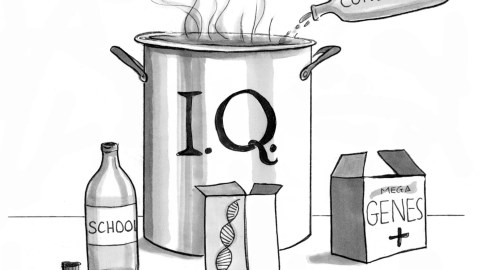
1. Do you know how genes work, or grasp what gene stats mean? A recurring IQ-and-genes fuss shows many are tempted into an epistemic comedy by seductive stats illusions, bad causology, and lax jargon.
2. IQ is 40-80% heritable and interracial IQ differences are “substantial [enough to]… affect… economic outcomes,” writes Andrew Sullivan, wielding science “bravely” alongside Sam Harris and Charles Murray.
3. Sullivan takes the data to mean individual IQ is 40-80% “caused by” genes. That’s not true, nor coherently knowable. Race-focused responses, like Ezra Klein’s, don’t explain the bonkers stats errors.
4. Technical heritability analyzes group variation stats not individual trait level factors. Stats professor Cosma Shalizi explains heritability “says nothing about how much [a trait’s level]… is under genetic control” and it’s “irrelevant to malleability” (heritable ≠ immutable; genes often aren’t carved-in-stone fate).
5. Exposing stats illusions Shalizi warns “causal-sounding phrases… encourage confusion” in many analyses of variance studies (where “due to,” “explained by,” “account for” don’t have ordinary meanings).
6. A not-known-enough thought experiment illustrates gene-nurture inseparability and misattribution risks—if society sent redheaded kids to bad schools, ginger genes would correlate with (seemingly “predict”) low IQ.
7. Many phenomena don’t fit the specific causal structure that basic stats presume—independent factors with additive effects. Most biological traits involve many, many gene-products playing hyper-complex, interdependent, non-additive roles via long intricate processes.
8. Statistically decomposing processes or functional systems is often like asking what percent of a car’s speed is caused by its engine or fuel or driver (all contribute inseparably).
9. How most genes work remains unknown, and causal opacity warrants extra caution. We do know genes often don’t work like switches or car parts, they’re more music-like.
10. Still, the “joy of stats” seduces many into confusion and causal overreach. For instance, Antonio Regalado covering dodgy DNA-to-IQ “predictors” uses “linked to” and “tied to” but slips into over-causal “explains” and “genetic determinants.”
11. Presumptive causality strains the statistical sense of sexy “polygenic scoring” methods, whose fans hope “predictions can operate in complete ignorance of the biological basis.” This data-fueled folly ignores “ginger-gene” complications.
12. Clearer-eyed experts offer cavernous caveats—neither heritability nor polygenic scoring illuminate genetic “causes” (Turkheimer), “summing” variant contributions isn’t wise (Racimo), and interpretation complexities abound (Novembre).
13. Lax causology and imprudent presumptive causality pervade genomics, “precision” medicine, Big Data and AI. The complete-ignorance-of-how fans forget that including non-causal factors skews, if not ruins, allocation-of-variation calculations (see “structured noise”).
14. Tread gingerly and always consider the causology. Are putative factors direct (“proximate”) causes, or many-complicated-steps removed? Is causal stability warranted? Or causal completeness? Does the causal structure fit stats tools? Do mixed response types muddy your metrics?
15. Correlation abuse and “multiple-regression monster” studies are as scandalous as science’s mass misuse of “statistical significance.”
16. Gene stats jargon has misled Sullivan (scientists like Harris and Murray have less excuse)—the “economic outcomes” leap gets the science, stats, and logic wrong (see Chris Dillow, Matthew Yglesias).