Few Maximize. Most Muddle. Modeling the Few Misleads the Many.
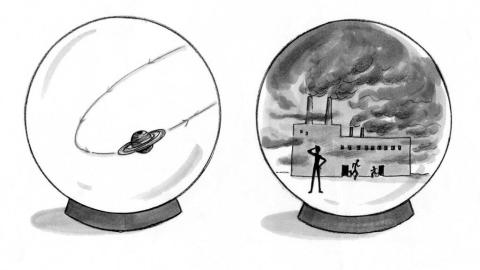
1. Few maximize. Most muddle. You know this; you muddle, or see many who do.
2. Yet economists mainly model the happy few. Perhaps, like the muddling masses, economists need less math, and more logic.
3. Maximization makes the math easier. This shapes the deep-metaphors, mathematical toolset, and even goals of many economists (justifying market as best allocator, at sacred equilibria).
4. Perhaps “people maximize” is a good modeling simplification (all models simplify)? As Richard Thaler’s American Economic Association president’s address says, we’re neither actual, nor “as if” maximizers.
5. Alan Greenspan in noting models, like maps, must exclude details, is unwittingly wise. Models peer into uncharted futures. But maps can’t cover the undiscovered.
6. Futures relate to pasts in at least three different, increasingly loose, ways — “Newton patterns,” “Darwin patterns,” and “Berlin patterns.”
7. The Newton pattern has universal laws, fixed interactions, tight causality —> detailed predictions.
8. The Darwin pattern has open, generative, universal processes, whose logic locally creates less fixed interactions and looser causalities. Patterns with choice + change + chance —> detailed outcomes are less predictable, less algebraically summarizable, they’re atomically algorithmic.
9. The Berlin pattern is less “nomothetic,” (nomos = law, thetic = generating). Isaiah Berlin felt history’s patterns could mutate quickly (=weakly nomothetic, moderately reliable maxims). Equivalent conditions don’t create equivalent results. History, like sports, plays less predictable games.
10. In Newton pattern domains, simplicity guides well, but empirically “nature often” resists Occam’s razor, “prefer[ing] complexity in biological and social” systems.
11. Back to economics, Paul Krugman justifies “equation filtering,” seeing no “rigorous way to model” even “obvious empirical” facts unless they’re in equations.
12. But having the equations ≠ having good models. Even Newton-pattern equations can create cloudy crystal balls — “We’re better at predicting what happens at the edge of the galaxy than … whether it’ll rain” (Tom Stoppard).
13. Economics has it way worse than the weather modellers. Economies are “complex adaptive systems” (less physic-like, more organism-like, or ecosystem-like).
13. Mark Thoma justifies economic “equilibrium shortcuts,” using frog-spacing strategies around a circular pond containing a snake. The equilibrium solution is simple: One bunched group maximizes survival. For Thoma the “enormous complexities” and umpteen generations getting to equilibrium are “irrelevant.” Not to those many unequilibrated generations.
14. Yes swifter economic adaptation —> faster equilibria, but also —> quicker disruption (there’s profit in pushing markets off equilibria).
15. Besides, equilibrium modeling can’t handle beehives, never mind economies (Herbert Gintis). Biologists don’t use math like physicists, (basically different patterns, microphysics can’t model ecosystems).
16. Algorithmic logic can rigorously model what Krugman’s equations can’t. “Agent-based modeling” can re-incorporate excluded behaviors — diverse, scripted logic to model muddlers (with rule-of-thumb maxims, and “cognitive biases,” = game-theoretic patterns).
17. Even if economics fit the Newton pattern, why expect it to beat weather forecasting? Its patterns are closer to evolution, or history, than to physics.
Miscasting us as prudent maximizers misdirects the massive power of markets (=muddled allocations).
Illustration by Julia Suits, The New Yorker Cartoonist & author of The Extraordinary Catalog of Peculiar Inventions.