How “centaur AI” will radically reshape the future of healthcare
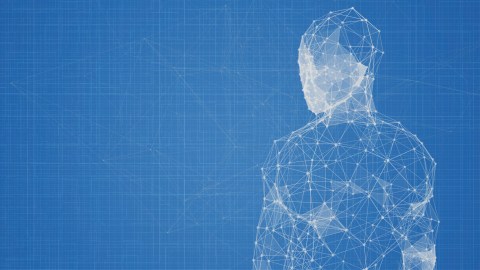
- Graphical processing units (GPUs) were instrumental in the advancement of deep learning networks.
- The “black box” nature of “deep nets” — logic we can’t fully comprehend — has huge diagnostic potential but critical limitations.
- With healthcare, it is not enough to spot patterns: we need to understand biological mechanisms.
AI systems are already transforming healthcare. Those changes will accelerate in the coming years to such a degree that AI will soon be as much a part of our healthcare experience as doctors, nurses, waiting rooms, and pharmacies. In fact, it won’t be long before AI has mostly replaced or redefined virtually all of these. As the dramatic expansion of telehealth during the COVID-19 pandemic has shown, when there is enough of a need, healthcare providers can pivot to adopt new strategies faster than we would imagine.
There are two different, yet complementary, approaches to AI. The first camp takes the view that, given enough data and computing power, we can derive complex models to accomplish difficult tasks—a great many, or possibly even all, of the tasks humans are capable of. The data camp believes that all we need is data and lots of computer cycles to solve problems. Domain expertise in the relevant area is not required. Want to get a computer to drive a car? With enough data, you can do that. Need a robot to bake a cake? Data will get you there. Wish to see a painting in the style of Berthe Morisot materialize before your very eyes? Data and massive computing power can do it.
The second camp bets on knowledge and focuses on imitating how humans actually reason, using conceptuality, connection, and causality. The knowledge camp believes in the critical requirement of domain expertise, building algorithms to apply approximations of accumulated human knowledge in order to execute logic on a fact pattern via what are commonly called expert systems. These are often rule-based or probabilistic calculations, such as if a patient’s HbA1c is higher than 6.5 percent and their fasting glucose is higher than 126 mg / dL, then there is a high likelihood that the patient has diabetes.
Today, data-driven AI is much further developed than knowledge-based AI, as the complexity of rules-based expert systems has been a significant impediment to scaling. The systems that enable self-driving cars to operate on our roads are all data-based. The algorithms that big tech companies use to guide ad placements, messaging, and recommendations are all data-based. As we will see, some important problems in biology are being solved brilliantly by data-driven AI, as well. But in an area as complex as human biology and disease, domain expertise may ultimately be more important in helping us make sense of the complex signal-to-noise issues that arise in big data. Indeed, it is likely that we will have to integrate the data-driven and knowledge-driven approaches to handle the extreme complexity of the human body.
Data are nothing without processing power. Neural network strategies have advanced enormously thanks to the demands of computer gaming, which provided the market forces that so often drive computational innovation. Gamers wanted realism and real-time responsiveness, and every advance toward these goals by one company stoked an arms race among others. It was in this hypercompetitive environment that graphical processing units, or GPUs, were developed to optimize the manipulation of images. If you’ve ever noticed how incredibly realistic video game characters and environments have become in recent years, you’re marveling at the hyperfast renderings made possible by GPUs.
These specialized electronic circuits didn’t stay in the realm of gaming for long. Andrew Ng, an AI leader and teacher of widely used online courses, was the first to recognize and exploit the power of GPUs to help neural networks bridge the gap between what the human brain evolved to do over millions of years and what computers have achieved over a matter of decades. He saw that the ultrafast matrix representations and manipulations made possible by GPUs were ideal for handling the hidden layers of input, processing, and output needed to create computer algorithms that could automatically improve themselves as they moved through the data. In other words, GPUs might help computers learn to learn.
Deep nets are great “analogizers.” They learn from what they see, but they can’t tell you about something new.
This was a big step forward. By Ng’s early estimates, GPUs could increase the speed of machine learning a hundredfold. Once this was coupled with fundamental advances in neural networks’ algorithms, such as backpropagation, led by luminaries like cognitive psychologist Geoffrey Hinton, we arrived in the age of “deep learning.”
What makes deep learning so deep? In the early days of artificial neural networks, the networks were shallow, often containing only a single “hidden layer” between the input data and the generated prediction. Now we have the ability to use artificial neural networks that are tens or even hundreds of layers deep, with each layer containing non-linear functions. Combine enough of these and you can represent arbitrarily complex relationships among data. As the number of layers has increased, so too has the capacity of these networks to discern patterns and make predictions from high-dimensional data. Correlating and integrating these features has been a game changer.
Consider what we could do by applying that sorting power to an individual’s personal data cloud. In goes the genome, phenome, digital measures of health, clinical data, and health status. Out come patterns recognized as indicative of early wellness-to-disease transitions and predictions of what choices might lie ahead with bifurcations in the disease trajectory (e.g., whether you could develop or avoid chronic kidney disease, or stave off advancing diabetes to regain metabolic health rather than progress to advanced stages with diabetic ulcers and foot amputations).
The potential is astonishing, but there are limitations to this approach. These high-quality predictions come from extremely complex functions, resulting in a “black box” that leads to a decision whose logic we can’t fully comprehend. Deep nets are great “analogizers.” They learn from what they see, but they can’t tell you about something new. Data-driven AI can help us find functions that fit trends in data. It can work virtual miracles when it comes to statistical prediction, with nuanced and accurate predictive capability. But it can do no more than that. And this is a critical distinction. A world where we based our understanding and actions on data correlation alone would be a very strange world indeed.
Computers are phenomenal at computing. What they’re not so good at is anything else.
How strange? Well, if you were to ask AI to tell you how to keep people from dying of chronic diseases, it is liable to tell you to murder the patient. Murder, after all, isn’t a chronic disease, and if done early in life, it would be 100 percent effective at ensuring no death from chronic disease. The sorts of options that are so ridiculous or immoral as to be inconceivable for most humans are on the table for computers because ridiculousness and immorality are human concepts that are not programmed into computers. It takes human programmers—presumably those with decency and compassion and a sense of ethics—to write specific lines of code limiting AI’s options. As Turing Award winner Judea Pearl put it in The Book of Why, “data are profoundly dumb.” Uberfast data are just profoundly dumb at light speed.
By “dumb,” Pearl didn’t mean “bad at what computers are supposed to do.” Of course not. Computers are phenomenal at computing. What they’re not so good at is anything else. Program a computer to play chess, and it can beat the greatest of human grand masters, but it won’t have any way of deciding the best use of its power after the game is over. And it isn’t aware that chess is a game or that it is playing a game.
This is something Garry Kasparov realized soon after his historic loss to IBM’s Deep Blue. Yes, the machine had defeated the man, but Kasparov would later note that, from his perspective, it seemed that many AI enthusiasts were rather disappointed. After all, they had long expected computers to overpower human competition; that much was inevitable. But “Deep Blue was hardly what their predecessors had imagined decades earlier,” Kasparov wrote. “Instead of a computer that thought and played chess like a human, with human creativity and intuition, they got one that played like a machine, systematically evaluating 200 million possible moves on the chess board per second and winning with brute number-crunching force.”
What happened next got far less press but was, to Kasparov, far more interesting. When he and other players didn’t compete with machines but instead teamed up with them, the human-plus-computer combination generally proved superior to the computer alone, chiefly because this melding of the minds changed their relationship to perceived risk. With the benefits of a computer able to run millions of permutations to prevent making a ruinous move or missing something obvious, human players could be freer to explore and engage in novel strategies, making them more creative and unpredictable in their play. This might not always be the case when it comes to games, which are closed systems where brute force and number-crunching ability are incredibly powerful, but we believe it is a vital lesson for twenty-first-century medicine, because, ultimately, when it comes to health, it is not enough to spot patterns: we need to understand biological mechanisms and to know why things happen as they do so that we can intervene appropriately.
The future of healthcare will take us to a place where increasing numbers of routine medical decisions are being made by AI alone. But far more decisions will come from a combined approach of powerful AI assessments augmented and amplified by highly trained human intelligence, a schema that has come to be known as “centaur AI.” Like the mythical half-human, half-horse creature of Greek mythology, this hybrid arrangement is part human, part computer and should offer us the best of both worlds. This is especially true in areas where extreme human complexities play major roles and brute computational power is likely to be less successful than it can be in a closed, fully specified system like a game.