No, AI did not discover a new type of physics
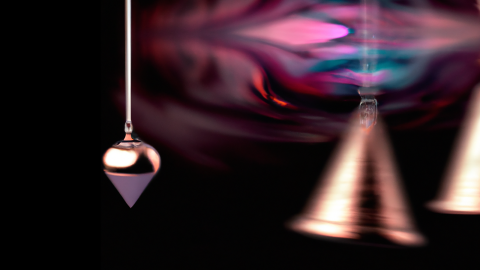
- First established by Isaac Newton, classical mechanics is a foundational field of physics.
- Recognizing the proper number of variables is key to solving its problems.
- Researchers have tested the ability of an “AI physicist” to accomplish this. At first, their result appeared promising; but on closer review, it is clearly a failure.
Can a computer algorithm discover something new about physics? It’s a fascinating question. A new research paper on the topic inspired the sensational headline “An AI may have just invented ‘alternative’ physics.”
The term “alternative physics” sounds a lot like “alternative facts,” but let’s investigate anyway. How does this computer program’s performance compare with that of an actual physicist? Or even that of an average student?
Newtonian mechanics
Isaac Newton was a peerless genius. The English polymath not only unified the studies of motion and gravity but invented the mathematical language with which to describe them. The concepts of classical mechanics brought into being by Newton underlie most of the physics invented since then. His concepts were subsequently reformulated in new mathematical language in the 18th century by the exceptional continental physicists Joseph-Louis Lagrange and Leonhard Euler.
Newton’s mechanics requires an analysis of the directional forces acting upon massive bodies. If you took an introductory high school or college physics class, you have seen these problems: boxes on inclined planes, pulleys, and carts. You draw arrows going in various directions and try to balance forces. It works nicely for small problems. As the problems become more complex, this method continues to work, but it becomes brutally tedious.
With Lagrange’s formulation, if two aspects of the nature of the system can be defined, the problem can be solved using only calculus. (Yes, “only” calculus: Crunching derivatives is much easier than solving extremely complex free-body diagrams where the arrows change at every position.)
The first thing to understand is the energy of the system, namely, the (kinetic) energy of movement and the (potential) energy stored by the configuration of the system. The second crucial thing is to choose proper coordinates, or variables, for the motion of the system.
Imagine a simple pendulum, like that in an old-fashioned clock. The pendulum bob has a kinetic energy from its swinging motion and a potential energy due to its position (height) within the gravitational field. The position of the pendulum can be described by a single variable: its angle relative to vertical. Lagrange’s solution for the pendulum’s motion can then be computed with relative ease.
Solving more complex problems in mechanics requires discovering the proper number of variables that can describe the system. In simple cases this is easy; in moderately complex cases, it is a student-level exercise. In extremely complex systems, it may be a professional’s work or impossible. This is where the AI “physicist” comes in.
AI physicist is beat by undergraduates
The computer was set to analyze the problem of a pendulum hanging on another pendulum. This problem requires two variables — the angle of each pendulum to the vertical — or four variables if a Cartesian (xy) coordinate system is used. If both pendulum bobs are hung from springs instead of rigid rods, the two variable spring lengths are added to get six variables in the Cartesian system.
The computer was asked to determine the number of variables needed to calculate the above problems. How did the AI physicist do? Not great. For the rigid pendulum on a pendulum, it gave two answers: ~7 and ~4-5. (The correct answer is 4 variables.) Similarly, it calculated ~8 and ~5-6 for the double-spring pendulum. (The correct answer is 6 variables.) The researchers praise the smaller estimates as being near the true answers.
But after digging into the details in the paper’s supplementary materials, however, the result begins to unravel. The computer didn’t actually calculate 4 variables and 6 variables. Its best calculations were 4.71 and 5.34. Neither of those answers even rounds to the correct answer. The four-variable problem is an intermediate undergraduate physics problem, while the six-variable problem is a more advanced undergraduate problem. In other words, an average undergraduate physics student is significantly better than the AI physicist at grasping these problems.
AI physicist is not ready for tenure
The researchers go on to ask the program to analyze complicated systems that not only have an unknown number of variables, but for which it is unclear whether classical mechanics can describe the systems at all. Examples include a lava lamp and fire. The AI does an acceptable job at predicting small changes in these systems. It also calculates the number of required variables (7.89 and 24.70, respectively). Correct answers to these problems would be “new physics,” in some sense, but there is no way of knowing if the AI is correct.
Using AI to analyze unknown systems is a neat idea, but the AI currently cannot get the easy answers right. Thus, we have no reason to believe it’s getting the hard ones right.