Are we in an AI summer or AI winter?
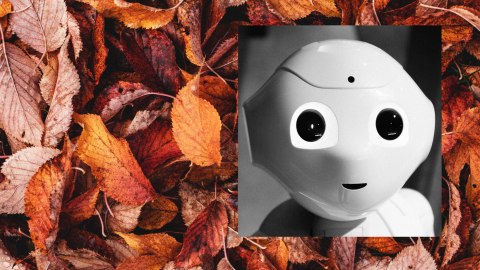
- The history of AI shows boom periods (AI summers) followed by busts (AI winters).
- The cyclical nature of AI funding is due to hype and promises not fulfilling expectations.
- This time, we might enter something resembling an AI autumn rather than an AI winter, but fundamental questions remain if true AI is even possible.
The dream of building a machine that can think like a human stretches back to the origins of electronic computers. But ever since research into artificial intelligence (AI) began in earnest after World War II, the field has gone through a series of boom and bust cycles called “AI summers” and “AI winters.”
Each cycle begins with optimistic claims that a fully, generally intelligent machine is just a decade or so away. Funding pours in and progress seems swift. Then, a decade or so later, progress stalls and funding dries up. Over the last ten years, we’ve clearly been in an AI summer as vast improvements in computing power and new techniques like deep learning have led to remarkable advances. But now, as we enter the third decade of the 21st century, some who follow AI feel the cold winds at their back leading them to ask, “Is Winter Coming?” If so, what went wrong this time?
How to build an A.I. brain that can conceive of itself | Joscha Bach | Big Thinkwww.youtube.comA brief history of AI
To see if the winds of winter are really coming for AI, it is useful to look at the field’s history. The first real summer can be pegged to 1956 and the famous Dartmouth University Workshop where one of the field’s pioneers, John McCarthy, coined the term “artificial intelligence.” The conference was attended by scientists like Marvin Minsky and H. A. Simon, whose names would go on to become synonymous with the field. For those researchers, the task ahead was clear: capture the processes of human reasoning through the manipulation of symbolic systems (i.e., computer programs).
Unless we are talking about very specific tasks, any 6-year-old is infinitely more flexible and general in his or her intelligence than the “smartest” Amazon robot.
Throughout the 1960s, progress seemed to come swiftly as researchers developed computer systems that could play chess, deduce mathematical theorems, and even engage in simple discussions with a person. Government funding flowed generously. Optimism was so high that, in 1970, Minsky famously proclaimed, “In three to eight years we will have a machine with the general intelligence of a human being.”
By the mid 1970s, however, it was clear that Minsky’s optimism was unwarranted. Progress stalled as many of the innovations of the previous decade proved too narrow in their applicability, seeming more like toys than steps toward a general version of artificial intelligence. Funding dried up so completely that researchers soon took pains not to refer to their work as AI, as the term carried a stink that killed proposals.
The cycle repeated itself in the 1980s with the rise of expert systems and the renewed interest in what we now call neural networks (i.e., programs based on connectivity architectures that mimic neurons in the brain). Once again, there was wild optimism and big increases in funding. What was novel in this cycle was the addition of significant private funding as more companies began to rely on computers as essential components of their business. But, once again, the big promises were never realized, and funding dried up again.
AI: Hype vs. reality
The AI summer we’re currently experiencing began sometime in the first decade of the new millennium. Vast increases in both computing speed and storage ushered in the era of deep learning and big data. Deep learning methods use stacked layers of neural networks that pass information to each other to solve complex problems like facial recognition. Big data provides these systems with vast oceans of examples (like images of faces) to train on. The applications of this progress are all around us: Google Maps give you near-perfect directions; you can talk with Siri anytime you want; IBM’s Deep Think computer beat Jeopardy’s greatest human champions.
In response, the hype rose again. True AI, we were told, must be just around the corner. In 2015, for example, The Guardianreported that self-driving cars, the killer app of modern AI, was close at hand. Readers were told, “By 2020 you will become a permanent backseat driver.” And just two years ago, Elon Musk claimed that by 2020 “we’d have over a million cars with full self-driving software.”
The general intelligence — i.e., the understanding — we humans exhibit may be inseparable from our experiencing. If that’s true, then our physical embodiment, enmeshed in a context-rich world, may be difficult if not impossible to capture in symbolic processing systems.
By now, it’s obvious that a world of fully self-driving cars is still years away. Likewise, in spite of the remarkable progress we’ve made in machine learning, we’re still far from creating systems that possess general intelligence. The emphasis is on the term general because that’s what AI really has been promising all these years: a machine that’s flexible in dealing with any situation as it comes up. Instead, what researchers have found is that, despite all their remarkable progress, the systems they’ve built remain brittle, which is a technical term meaning “they do very wrong things when given unexpected inputs.” Try asking Siri to find “restaurants that aren’t McDonald’s.” You won’t like the results.
Unless we are talking about very specific tasks, any 6-year-old is infinitely more flexible and general in his or her intelligence than the “smartest” Amazon robot.
Even more important is the sense that, as remarkable as they are, none of the systems we’ve built understand anything about what they are doing. As philosopher Alva Noe said of Deep Think’s famous Jeopardy! victory, “Watson answered no questions. It participated in no competition. It didn’t do anything. All the doing was on our side. We played Jeapordy! with Watson.” Considering this fact, some researchers claim that the general intelligence — i.e., the understanding — we humans exhibit may be inseparable from our experiencing. If that’s true, then our physical embodiment, enmeshed in a context-rich world, may be difficult if not impossible to capture in symbolic processing systems.
Not the (AI) winter of our discontent
Thus, talk a of a new AI winter is popping up again. Given the importance of deep learning and big data in technology, it’s hard to imagine funding for these domains drying up any time soon. What we may be seeing, however, is a kind of AI autumn when researchers wisely recalibrate their expectations and perhaps rethink their perspectives.