Algorithms identify repeat offenders better than judges
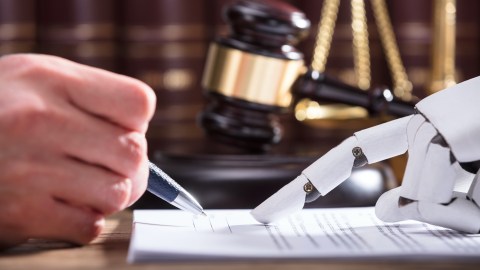
Image source: Andrey_Popov/Shutterstock
- A new study finds algorithmic predictions of recidivism more accurate than human authorities.
- Researchers are trying to construct tests of such AI that accurately mirror real-world deliberations.
- What level of reliability should we demand of AI in sentencing?
It’s pre-crime time again. (See Minority Report.)
When judges, correctional authorities, and parole boards are making sentencing, supervision, and release decisions, they’re essentially trying to peer into an offender’s future to assess the person’s potential for recidivism. To help guide these determinations — and no doubt influenced by our contemporary infatuation with artificial intelligence — authorities are increasingly turning to risk assessment instruments (RAIs) on the assumption that their AI can more accurately identify those likely to be repeat offenders.
A new study in Science Advances more rigorously confirms that algorithmic judgements may in fact be more accurate than humans. Of concern, though, is that given the stakes involved — future crimes, a defendant’s freedom or continued incarceration — they’re still not reliable enough to ensure that justice is truly done and that tragic mistakes can be avoided.
Image source: Andrey Suslov/Shutterstock
RAIs, NG?
The new study, led by computational social scientist Sharad Goel of Stanford University, is in a sense a reply to a recent work by programming expert Julia Dressel and digital image specialist Hany Farid. In that earlier research, participants attempted to predict whether or not any of 50 individuals would commit new crimes of any kind within the next two years based on short descriptions of their case histories. (No images or racial/ethnic information were provided to participants to avoid a skewing of results due to related biases.) The average accuracy rate participants achieved was 62%.
The same criminals and case histories cases were also processed through a widely used RAI called COMPAS, for “Correctional Offender Management Profiling for Alternative Sanctions.” The accuracy of its predictions was about the same: 65%, leading Dressel and Farid to conclude that COMPAS “is no more accurate … than predictions made by people with little or no criminal justice expertise.”
Taking a second look
Goel felt that two aspects of the testing method used by Dressel and Farid didn’t reproduce closely enough the circumstances in which humans are called upon to predict recidivism during sentencing:
- Participants in that study learned how to improve their predictions, much as an algorithm might, as they were provided feedback as to the accuracy of each prognostication. However, as Goel points out, “In justice settings, this feedback is exceedingly rare. Judges may never find out what happens to individuals that they sentence or for whom they set bail.”
- Judges, etc. also often have a great deal of information in hand as they make their predictions, not short summaries in which only the most salient information is presented. In the real world, it can be hard to ascertain which information is the most relevant when there’s arguably too much of it at hand.
Both of these factors put participants on a more equal footing with an RAI than they would be in real life, perhaps accounting for the similar levels of accuracy encountered.
To that end, Goel and his colleagues performed several of their own, slightly different, trials.
The first experiment closely mirrored Dressel’s and Farid’s — with feedback and short case descriptions — and indeed found that humans and COMPAS performed pretty much equally well. Another experiment asked participants to predict the future occurrence of violent crime, not just any crime, and again the accuracy rates were comparable, though much higher. Humans scored 83% as COMPAS achieved 89% accuracy.
When participant feedback was removed, however, humans fell far behind COMPAS in accuracy, down to around 60% as opposed to COMPAS’s 89%, as Goel hypothesized they might.
Finally, humans were tested against a different RAI tool called LSI-R. In this case, both had to try and predict an individual’s future using on a large amount of case information similar to what a judge may have to wade through. Again, the RAI outperformed humans in predicting future crimes, 62% to 57%. When asked to predict who would wind up going back to prison for their future misdeeds, the results were even worse for participants, who got it right just 58% of the time as opposed to 74% for LSI-R.
Image source: klss/Shutterstock
Good enough?
Goel concludes, “our results support the claim that algorithmic risk assessments can often outperform human predictions of reoffending.” Of course, this isn’t the only important question. There’s also this: Is AI yet reliable enough to make its prediction count for more than that of a judge, correctional authority, or parole board member?
Science News asked Farid, and he said no. When asked how he’d feel about an RAI that could be counted on to be right 80% of the time, he responded, “you’ve got to ask yourself, if you’re wrong 20 percent of the time, are you willing to tolerate that?”
As AI technology improves, we may one day reach a state in which RAIs are reliably accurate, but no one is claiming we’re there yet. For now, then, the use of such technologies in an advisory role for authorities tasked with making sentencing decisions may make sense, but only as one more “voice” to consider.