Why A.I. is a big fat lie
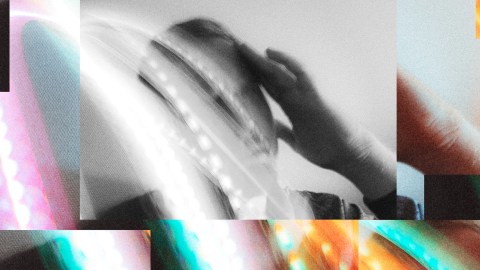
- All the hype around artificial intelligence misunderstands what intelligence really is.
- And A.I. is definitely, definitely not going to kill you, ever.
- Machine learning as a process and a concept, however, holds more promise.
A.I. is a big fat lie
A.I. is a big fat lie. Artificial intelligence is a fraudulent hoax — or in the best cases it’s a hyped-up buzzword that confuses and deceives. The much better, precise term would instead usually be machine learning – which is genuinely powerful and everyone oughta be excited about it.
On the other hand, AI does provide some great material for nerdy jokes. So put on your skepticism hat, it’s time for an AI-debunkin’, slam-dunkin’, machine learning-lovin’, robopocalypse myth-bustin’, smackdown jamboree – yeehaw!
3 main points
1) Unlike AI, machine learning’s totally legit. I gotta say, it wins the Awesomest Technology Ever award, forging advancements that make ya go, “Hooha!”. However, these advancements are almost entirely limited to supervised machine learning, which can only tackle problems for which there exist many labeled or historical examples in the data from which the computer can learn. This inherently limits machine learning to only a very particular subset of what humans can do – plus also a limited range of things humans can’t do.
2) AI is BS. And for the record, this naysayer taught the Columbia University graduate-level “Artificial Intelligence” course, as well as other related courses there.
AI is nothing but a brand. A powerful brand, but an empty promise. The concept of “intelligence” is entirely subjective and intrinsically human. Those who espouse the limitless wonders of AI and warn of its dangers – including the likes of Bill Gates and Elon Musk – all make the same false presumption: that intelligence is a one-dimensional spectrum and that technological advancements propel us along that spectrum, down a path that leads toward human-level capabilities. Nuh uh. The advancements only happen with labeled data. We are advancing quickly, but in a different direction and only across a very particular, restricted microcosm of capabilities.
The term artificial intelligence has no place in science or engineering. “AI” is valid only for philosophy and science fiction – and, by the way, I totally love the exploration of AI in those areas.
3) AI isn’t gonna kill you. The forthcoming robot apocalypse is a ghost story. The idea that machines will uprise on their own volition and eradicate humanity holds no merit.
Neural networks for the win
In the movie “Terminator 2: Judgment Day,” the titular robot says, “My CPU is a neural net processor, a learning computer.” The neural network of which that famous robot speaks is actually a real kind of machine learning method. A neural network is a way to depict a complex mathematical formula, organized into layers. This formula can be trained to do things like recognize images for self-driving cars. For example, watch several seconds of a neural network performing object recognition.
What you see it doing there is truly amazing. The network’s identifying all those objects. With machine learning, the computer has essentially programmed itself to do this. On its own, it has worked out the nitty gritty details of exactly what patterns or visual features to look for. Machine learning’s ability to achieve such things is awe-inspiring and extremely valuable.
The latest improvements to neural networks are called deep learning. They’re what make this level of success in object recognition possible. With deep learning, the network is quite literally deeper – more of those layers. However, even way way back in 1997, the first time I taught the machine learning course, neural networks were already steering self-driving cars, in limited contexts, and we even had our students apply them for face recognition as a homework assignment.
The achitecture for a simple neural network with four layers
But the more recent improvements are uncanny, boosting its power for many industrial applications. So, we’ve even launched a new conference, Deep Learning World, which covers the commercial deployment of deep learning. It runs alongside our long-standing machine learning conference series, Predictive Analytics World.
Supervised machine learning requires labeled data
So, with machines just getting better and better at humanlike tasks, doesn’t that mean they’re getting smarter and smarter, moving towards human intelligence?
No. It can get really, really good at certain tasks, but only when there’s the right data from which to learn. For the object recognition discussed above, it learned to do that from a large number of example photos within which the target objects were already correctly labeled. It needed those examples to learn to recognize those kinds of objects. This is called supervised machine learning: when there is pre-labeled training data. The learning process is guided or “supervised” by the labeled examples. It keeps tweaking the neural network to do better on those examples, one incremental improvement at a time. That’s the learning process. And the only way it knows the neural network is improving or “learning” is by testing it on those labeled examples. Without labeled data, it couldn’t recognize its own improvements so it wouldn’t know to stick with each improvement along the way. Supervised machine learning is the most common form of machine learning.
Here’s another example. In 2011, IBM’s Watson computer defeated the two all-time human champions on the TV quiz show Jeopardy. I’m a big fan. This was by far the most amazing thing I’ve seen a computer do – more impressive than anything I’d seen during six years of graduate school in natural language understanding research. Here’s a 30-second clip of Watson answering three questions.
To be clear, the computer didn’t actually hear the spoken questions but rather was fed each question as typed text. But its ability to rattle off one answer after another – given the convoluted, clever wording of Jeopardy questions, which are designed for humans and run across any and all topics of conversation – feels to me like the best “intelligent-like” thing I’ve ever seen from a computer.
But the Watson machine could only do that because it had been given many labeled examples from which to learn: 25,000 questions taken from prior years of this TV quiz show, each with their own correct answer.
At the core, the trick was to turn every question into a yes/no prediction: “Will such-n-such turn out to be the answer to this question?” Yes or no. If you can answer that question, then you can answer any question – you just try thousands of options out until you get a confident “yes.” For example, “Is ‘Abraham Lincoln’ the answer to ‘Who was the first president?'” No. “Is ‘George Washington’?” Yes! Now the machine has its answer and spits it out.
Computers that can talk like humans
And there’s another area of language use that also has plentiful labeled data: machine translation. Machine learning gobbles up a feast of training data for translating between, say, English and Japanese, because there are tons of translated texts out there filled with English sentences and their corresponding Japanese translations.
In recent years, Google Translate – which anyone can use online – swapped out the original underlying solution for a much-improved one driven by deep learning. Go try it out – translate a letter to your friend or relative who has a different first language than you. I use it a lot myself.
On the other hand, general competence with natural languages like English is a hallmark of humanity – and only humanity. There’s no known roadmap to fluency for our silicon sisters and brothers. When we humans understand one another, underneath all the words and somewhat logical grammatical rules is “general common sense and reasoning.” You can’t work with language without that very particular human skill. Which is a broad, unwieldy, amorphous thing we humans amazingly have.
So our hopes and dreams of talking computers are dashed because, unfortunately, there’s no labeled data for “talking like a person.” You can get the right data for a very restricted, specific task, like handling TV quiz show questions, or answering the limited range of questions people might expect Siri to be able to answer. But the general notion of “talking like a human” is not a well-defined problem. Computers can only solve problems that are precisely defined.
So we can’t leverage machine learning to achieve the typical talkative computer we see in so many science fiction movies, like the Terminator, 2001‘s evil HAL computer, or the friendly, helpful ship computer in Star Trek. You can converse with those machines in English very much like you would with a human. It’s easy. Ya just have to be a character in a science fiction movie.
Intelligence is subjective, so A.I. has no real definition
Now, if you think you don’t already know enough about AI, you’re wrong. There is nothing to know, because it isn’t actually a thing. There’s literally no meaningful definition whatsoever. AI poses as a field, but it’s actually just a fanciful brand. As a supposed field, AI has many competing definitions, most of which just boil down to “smart computer.” I must warn you, do not look up “self-referential” in the dictionary. You’ll get stuck in an infinite loop.
Many definitions are even more circular than “smart computer,” if that’s possible. They just flat out use the word “intelligence” itself within the definition of AI, like “intelligence demonstrated by a machine.”
If you’ve assumed there are more subtle shades of meaning at hand, surprise – there aren’t. There’s no way to resolve how utterly subjective the word “intelligence” is. For computers and engineering, “intelligence” is an arbitrary concept, irrelevant to any precise goal. All attempts to define AI fail to solve its vagueness.
Now, in practice the word is often just – confusingly – used as a synonym for machine learning. But as for AI as its own concept, most proposed definitions are variations of the following three:
1) AI is getting a computer to think like a human. Mimic human cognition. Now, we have very little insight into how our brains pull off what they pull off. Replicating a brain neuron-by-neuron is a science fiction “what if” pipe dream. And introspection – when you think about how you think – is interesting, big time, but ultimately tells us precious little about what’s going on in there.
2) AI is getting a computer to act like a human. Mimic human behavior. Cause if it walks like a duck and talks like a duck… But it doesn’t and it can’t and we’re way too sophisticated and complex to fully understand ourselves, let alone translate that understanding into computer code. Besides, fooling people into thinking a computer in a chatroom is actually a human – that’s the famous Turing Test for machine intelligence – is an arbitrary accomplishment and it’s a moving target as we humans continually become wiser to the trickery used to fool us.
3) AI is getting computers to solve hard problems. Get really good at tasks that seem to require “intelligence” or “human-level” capability, such as driving a car, recognizing human faces, or mastering chess. But now that computers can do them, these tasks don’t seem so intelligent after all. Everything a computer does is just mechanical and well understood and in that way mundane. Once the computer can do it, it’s no longer so impressive and it loses its charm. A computer scientist named Larry Tesler suggested we define intelligence as “whatever machines haven’t done yet.” Humorous! A moving-target definition that defines itself out of existence.
By the way, the points in this article also apply to the term “cognitive computing,” which is another poorly-defined term coined to allege a relationship between technology and human cognition.
The logical fallacy of believing in A.I.’s innevitability
The thing is, “artificial intelligence” itself is a lie. Just evoking that buzzword automatically insinuates that technological advancement is making its way toward the ability to reason like people. To gain humanlike “common sense.” That’s a powerful brand. But it’s an empty promise. Your common sense is more amazing – and unachievable – than your common sense can sense. You’re amazing. Your ability to think abstractly and “understand” the world around you might feel simple in your moment-to-moment experience, but it’s incredibly complex. That experience of simplicity is either a testament to how adept your uniquely human brain is or a great illusion that’s intrinsic to the human condition – or probably both.
Now, some may respond to me, “Isn’t inspired, visionary ambition a good thing? Imagination propels us and unknown horizons beckon us!” Arthur C. Clarke, the author of 2001, made a great point: “Any sufficiently advanced technology is indistinguishable from magic.” I agree. However, that does not mean any and all “magic” we can imagine – or include in science fiction – could eventually be achieved by technology. Just ’cause it’s in a movie doesn’t mean it’s gonna happen. AI evangelists often invoke Arthur’s point – but they’ve got the logic reversed. My iPhone seems very “Star Trek” to me, but that’s not an argument everything on Star Trek is gonna come true. The fact that creative fiction writers can make shows like Westworld is not at all evidence that stuff like that could happen.
Now, maybe I’m being a buzzkill, but actually I’m not. Let me put it this way. The uniqueness of humans and the real advancements of machine learning are each already more than amazing and exciting enough to keep us entertained. We don’t need fairy tales – especially ones that mislead.
Sophia: A.I.’s most notoriously fraudulent publicity stunt
The star of this fairy tale, the leading role of “The Princess” is played by Sophia, a product of Hanson Robotics and AI’s most notorious fraudulent publicity stunt. This robot has applied her artificial grace and charm to hoodwink the media. Jimmy Fallon and other interviewers have hosted her – it, I mean have hosted it. But when it “converses,” it’s all scripts and canned dialogue – misrepresented as spontaneous conversation – and in some contexts, rudimentary chatbot-level responsiveness.
Believe it or not, three fashion magazines have featured Sophia on their cover, and, ever goofier and sillier, the country Saudi Arabia officially granted it citizenship. For real. The first robot citizen. I’m actually a little upset about this, ’cause my microwave and pet rock have also applied for citizenship but still no word.
Sophia is a modern-day Mechanical Turk – which was an 18th century hoax that fooled the likes of Napoleon Bonaparte and Benjamin Franklin into believing they’d just lost a game of chess to a machine. A mannequin would move the chess pieces and the victims wouldn’t notice there was actually a small human chess expert hidden inside a cabinet below the chess board.
In a modern day parallel, Amazon has an online service you use to hire workers to perform many small tasks that require human judgement, like choosing the nicest looking of several photographs. It’s named Amazon Mechanical Turk, and its slogan, “Artificial Artificial Intelligence.” Which reminds me of this great vegetarian restaurant with “mock mock duck” on the menu – I swear, it tastes exactly like mock duck. Hey, if it talks like a duck, and it tastes like a duck…
Yes indeed, the very best fake AI is humans. In 1965, when NASA was defending the idea of sending humans to space, they put it this way: “Man is the lowest-cost, 150-pound, nonlinear, all-purpose computer system which can be mass-produced by unskilled labor.” I dunno. I think there’s some skill in it. 😉
The myth of dangerous superintelligence
Anyway, as for Sophia, mass hysteria, right? Well, it gets worse: Claims that AI presents an existential threat to the human race. From the most seemingly credible sources, the most elite of tech celebrities, comes a doomsday vision of homicidal robots and killer computers. None other than Bill Gates, Elon Musk, and even the late, great Stephen Hawking have jumped on the “superintelligence singularity” bandwagon. They believe machines will achieve a degree of general competence that empowers the machines to improve their own general competence – so much so that this will then quickly escalate past human intelligence, and do so at the lightning speed of computers, a speed the computers themselves will continue to improve by virtue of their superintelligence, and before you know it you have a system or entity so powerful that the slightest misalignment of objectives could wipe out the human race. Like if we naively commanded it to manufacture as many rubber chickens as possible, it might invent an entire new high-speed industry that can make 40 trillion rubber chickens but that happens to result in the extinction of Homo sapiens as a side effect. Well, at least it would be easier to get tickets for Hamilton.
There are two problems with this theory. First, it’s so compellingly dramatic that it’s gonna ruin movies. If the best bad guy is always a robot instead of a human, what about Nurse Ratched and Norman Bates? I need my Hannibal Lecter! “The best bad guy,” by the way, is an oxymoron. And so is “artificial intelligence.” Just sayin’.
But it is true: Robopocalypse is definitely coming. Soon. I’m totally serious, I swear. Based on a novel by the same name, Michael Bay – of the “Transformers” movies – is currently directing it as we speak. Fasten your gosh darn seatbelts people, ’cause, if “Robopocalypse” isn’t in 3D, you were born in the wrong parallel universe.
Oh yeah, and the second problem with the AI doomsday theory is that it’s ludicrous. AI is so smart it’s gonna kill everyone by accident? Really really stupid superintelligence? That sounds like a contradiction.
To be more precise, the real problem is that the theory presumes that technological advancements move us along a path toward humanlike “thinking” capabilities. But they don’t. It’s not headed in that direction. I’ll come back to that point again in a minute – first, a bit more on how widely this apocalyptic theory has radiated.
A widespread belief in superintelligence
The Kool-Aid these high-tech royalty drink, the go-to book that sets the foundation, is the New York Times bestseller “Superintelligence,” by Nick Bostrom, who’s a professor of applied ethics at Oxford University. The book mongers the fear and fans the flames, if not igniting the fire in the first place for many people. It explores how we might “make an intelligence explosion survivable.” The Guardian newspaper ran a headline, “Artificial intelligence: ‘We’re like children playing with a bomb’,” and Newsweek: “Artificial Intelligence Is Coming, and It Could Wipe Us Out,” both headlines obediently quoting Bostrom himself.
Bill Gates “highly recommends” the book, Elon Musk said AI is “vastly more risky than North Korea” – as Fortune Magazine repeated in a headline – and, quoting Stephen Hawking, the BBC ran a headline, “‘AI could spell end of the human race’.”
In a Ted talk that’s been viewed 5 million times (across platforms), the bestselling author and podcast intellectual Sam Harris states with supreme confidence, “At a certain point, we will build machines that are smarter than we are, and once we have machines that are smarter than we are, they will begin to improve themselves.”
Both he and Bostrom show the audience an intelligence spectrum during their Ted talks – here’s the one by Bostrom:
What happens when our computers get smarter than we are? | Nick Bostrom
You can see as we move along the path from left to right we pass a mouse, a chimp, a village idiot, and then the very smart theoretical physicist Ed Witten. He’s relatively close to the idiot, because even an idiot human is much smarter than a chimp, relatively speaking. You can see the arrow just above the spectrum showing that “AI” progresses in that same direction, along to the right. At the very rightmost position is Bostrom himself, which is either just an accident of photography, or proof that he himself is an AI robot.
In fact, here’s a 13-second clip of the moment that Bill Gates first brought Bostrom to life.
Oops, that was the wrong clip – uh, that was Dr. Frankenstein, but, ya know, same scenario.
A falsely conceived “spectrum of intelligence”
Anyway, that falsely-conceived intelligence spectrum is the problem. I’ve read the book and many of the interviews and watched the talks and pretty much all the believers intrinsically build on an erroneous presumption that “smartness” or “intelligence” falls more or less along a single, one-dimensional spectrum. They presume that the more adept machines become at more and more challenging tasks, the higher they will rank on this scale, eventually surpassing humans.
But machine learning has us marching along a different path. We’re moving fast, and we’ll likely go very far, but we’re going in a different direction, only tangentially related to human capabilities.
The trick is to take a moment to think about this difference. Our own personal experiences of being one of those smart creatures called a human is what catches us in a thought trap. Our very particular and very impressive capabilities are hidden from ourselves beneath a veil of a conscious experience that just kind of feels like “clarity.” It feels simple, but under the surface, it’s oh so complex. Replicating our “general common sense” is a fanciful notion that no technological advancements have ever moved us towards in any meaningful way.
Thinking abstractly often feels uncomplicated. We draw visuals in our mind, like a not-to-scale map of a city we’re navigating, or a “space” of products that two large companies are competing to sell, with each company dominating in some areas but not in others… or, when thinking about AI, the mistaken vision that increasingly adept capabilities – both intellectual and computational – all fall along the same, somewhat narrow path.
Now, Bostrom rightly emphasizes that we should not anthropomorphize what intelligent machines may be like in the future. It’s not human, so it’s hard to speculate on the specifics and perhap it will seem more like a space alien’s intelligence. But what Bostrom and his followers aren’t seeing is that, since they believe technology advances along a spectrum that includes and then transcends human cognition, the spectrum itself as they’ve conceived it is anthropomorphic. It has humanlike qualities built in. Now, your common sense reasoning may seem to you like a “natural stage” of any sort of intellectual development, but that’s a very human-centric perspective. Your common sense is intricate and very, very particular. It’s far beyond our grasp – for anyone – to formally define a “spectrum of intelligence” that includes human cognition on it. Our brains are spectacularly multi-faceted and adept, in a very arcane way.
Machines progress along a different spectrum
Machine learning actually does work by defining a kind of spectrum, but only for an extremely limited sort of trajectory – only for tasks that have labeled data, such as identifying objects in images. With labeled data, you can compare and rank various attempts to solve the problem. The computer uses the data to measure how well it does. Like, one neural network might correctly identify 90% of the trucks in the images and then a variation after some improvements might get 95%.
Getting better and better at a specific task like that obviously doesn’t lead to general common sense reasoning capabilities. We’re not on that trajectory, so the fears should be allayed. The machine isn’t going to get to a human-like level where it then figures out how to propel itself into superintelligence. No, it’s just gonna keep getting better at identifying objects, that’s all.
Intelligence isn’t a Platonic ideal that exists separately from humans, waiting to be discovered. It’s not going to spontaneously emerge along a spectrum of better and better technology. Why would it? That’s a ghost story.
It might feel tempting to believe that increased complexity leads to intelligence. After all, computers are incredibly general-purpose – they can basically do any task, if only we can figure out how to program them to do that task. And we’re getting them to do more and more complex things. But just because they could do anything doesn’t mean they will spontaneously do everything we imagine they might.
No advancements in machine learning to date have provided any hint or inkling of what kind of secret sauce could get computers to gain “general common sense reasoning.” Dreaming that such abilities could emerge is just wishful thinking and rogue imagination, no different now, after the last several decades of innovations, than it was back in 1950, when Alan Turing, the father of computer science, first tried to define how the word “intelligence” might apply to computers.
Don’t sell, buy, or regulate on A.I.
Machines will remain fundamentally under our control. Computer errors will kill – people will die from autonomous vehicles and medical automation – but not on a catastrophic level, unless by the intentional design of human cyber attackers. When a misstep does occur, we take the system offline and fix it.
Now, the aforementioned techno-celebrity believers are true intellectuals and are truly accomplished as entrepreneurs, engineers, and thought leaders in their respective fields. But they aren’t machine learning experts. None of them are. When it comes to their AI pontificating, it would truly be better for everyone if they published their thoughts as blockbuster movie scripts rather than earnest futurism.
It’s time for term “AI” to be “terminated.” Mean what you say and say what you mean. If you’re talking about machine learning, call it machine learning. The buzzword “AI” is doing more harm than good. It may sometimes help with publicity, but to at least the same degree, it misleads. AI isn’t a thing. It’s vaporware. Don’t sell it and don’t buy it.
And most importantly, do not regulate on “AI”! Technology greatly needs regulation in certain arenas, for example, to address bias in algorithmic decision-making and the development of autonomous weapons – which often use machine learning – so clarity is absolutely critical in these discussions. Using the imprecise, misleading term “artificial intelligence” is gravely detrimental to the effectiveness and credibility of any initiative that regulates technology. Regulation is already hard enough without muddying the waters.
Want more of Dr. Data?
Click here to view more episodes and to sign up for future episodes of The Dr. Data Show.