The wild evolution of data science and how to unpack it
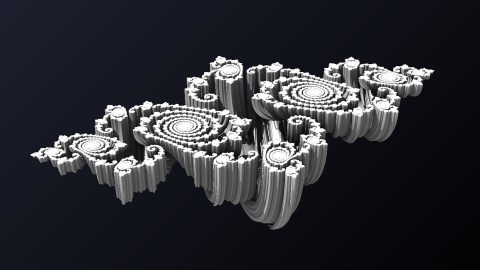
- Definitions of data science span a contentiously broad range.
- In academia, data science includes the messiness of “data janitorial work” and the subtleties of communicating results through data.
- Most arguments over the definition of data science come down to power and funding.
“I saw the best minds of my generation destroyed by madness,” wrote the poet Allen Ginsberg. In clause after clause, Ginsberg sang of the gulf between higher aspiration and the realities of Cold War America: “angelheaded hipsters burning for the ancient heavenly connection to the starry dynamo in the machinery of night” — and the chasm experienced by students with the increasingly militarized universities: “who passed through universities with radiant cool eyes hallucinating Arkansas and Blake-light tragedy among the scholars of war.”
In 2011, Jeff Hammerbacher, a former Facebook data team leader, riffing on Ginsberg, bemoaned, “The best minds of my generation are thinking about how to make people click ads. That sucks.” Of all the things to optimize, a generation had chosen manipulating attention.
Along with DJ Patil, Hammerbacher is credited with coining the term “data scientist” to describe a crucial new role in the corporate world from start-ups to Fortune 500 corporations. What does a data scientist do differently than practitioners of all the various quantitative approaches to the world we’ve seen? What exactly is “data science”? Definitions, we will see, vary.
Industrial data science came to mean machine learning and statistics combined with the software engineering and concrete data work needed to build digital products and services. In academic research, the term is capacious, extending beyond statistics to include the broader and less “technical” skills needed for making sense of the world through data, from the messiness of “data janitorial work” to the nuances of communicating results through data. Rather than abstractly “burning for the ancient heavenly connection,” the term speaks to the practical complexities of such work, starting with data analysis getting grubby with data. Riffing on Robert A. Heinlein, a very different Cold War writer, the data scientist Joel Grus satirized the expectation a “data scientist” had mastered the wide diversity of data tasks needed in industry:
“a data scientist should be able to run a regression, write a sql query, scrape a web site, design an experiment, factor matrices, use a data frame, pretend to understand deep learning, steal from the d3 gallery, argue r versus python, think in mapreduce, update a prior, build a dashboard, clean up messy data, test a hypothesis, talk to a businessperson, script a shell, code on a whiteboard, hack a p-value, machine-learn a model. specialization is for engineers.”
As the field rose to prominence in industry and academia, with associated job opportunities, funding opportunities, and new departments and degrees, employers and administrators sought to define things more precisely. Often, trying to nail down “data science” devolves into a verbal tussle in the online comment sections which coevolved with the internet. Rather than insist on one definition of “data science,” we seek to outline contours of contestation around the term.
Making sense of the world through data had been transformational.
For a decade now, in presentations, through memes, in comments to posts, practitioners have fought over what the term really stands for, in contrast to say statistics, machine learning, or earlier “data mining.” The arguments fundamentally concern who has authority and who gains capacities to rearrange power in dealing with data. And they concern who ultimately gets the funding — in corporations, in academia, and from the government.
To be clear, there was good reason for excitement and funding. In a variety of industries, making sense of the world through data had been transformational. The ability to recommend the right product and content to commercial users made possible a so-called “long tail” business model.
Similarly, in commercial software, we’ve become used to phones as devices we can talk “to,” not “on,” as speech recognition has improved through multiple quantum leaps. In finance, the single most profitable fund, the Medallion Fund at Renaissance Technologies, trades using statistical analysis, along with considerable attention to the software engineering needed to gather data, learn models, and execute trades.
In biology and human health, it was quickly realized that the sequencing of whole genomes in the 1990s had the potential to change our understanding of complex human diseases through data. “Biology is in the midst of an intellectual and experimental sea change,” declared the biologist Shirley Tilghman in the first sentence of an article in Nature in 2000. “Essentially the discipline is moving from being largely a data-poor science to becoming a data-rich science.”
In a wide variety of fields of human endeavor, it was clear that “new technology permitted entirely new questions,” that “will require . . . new sets of analytical tools.”