I’m a rational optimist. Here’s why I don’t believe in an AI doomsday.
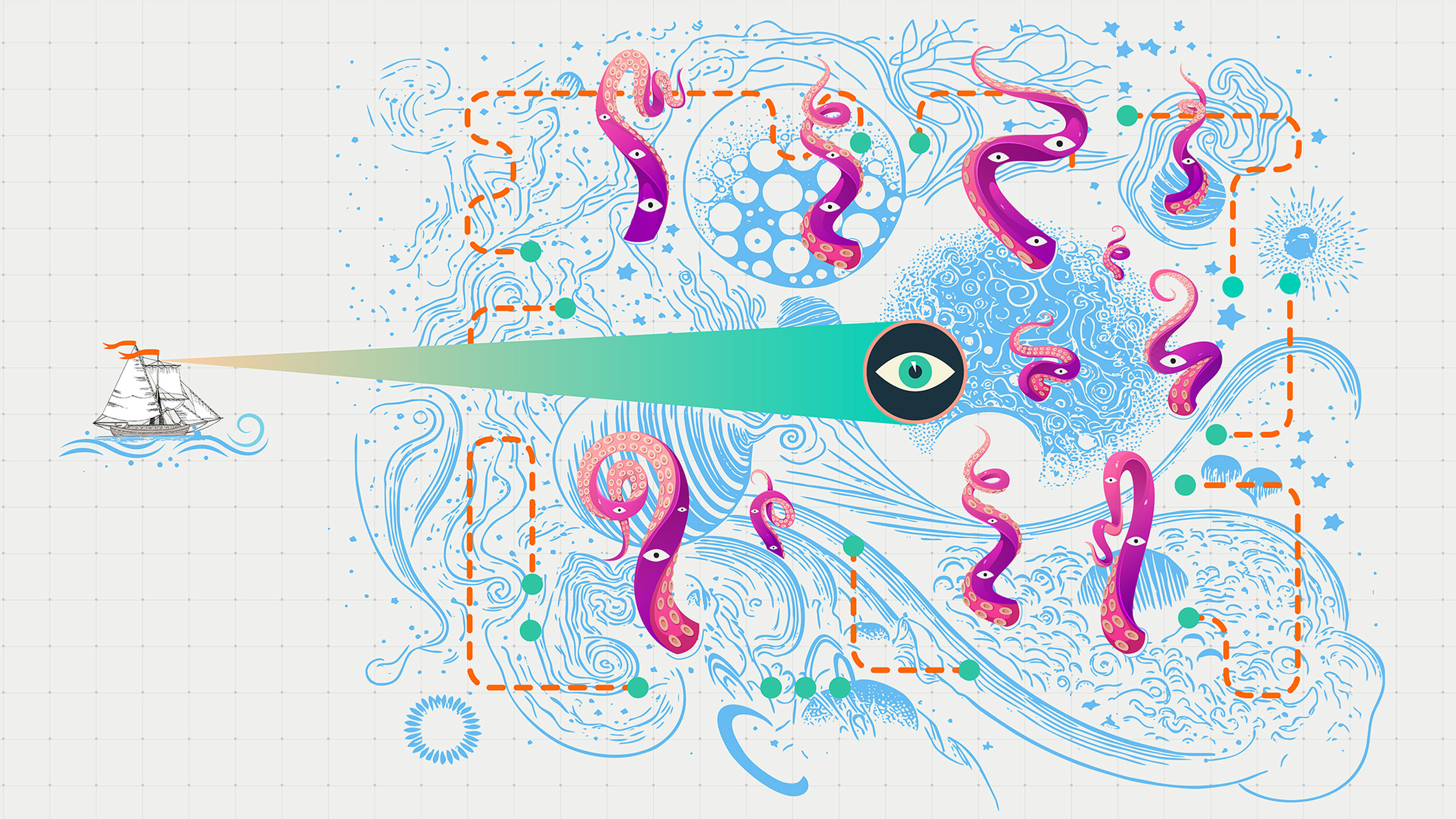
We live in interesting times. The systems of today are powerful. They can write, paint, direct, plan, code, and even write passable prose. And with this explosion of capabilities, we also have an explosion of worries. In seeing some of these current problems and projecting them into future non-extant problems, we find ourselves in a bit of a doom loop. The more fanciful arguments about how artificial superintelligence is inevitable and how they’re incredibly dangerous sit side by side with more understandable concerns about increasing misinformation.
It’s as if we’ve turned the world into a chess game. In chess, you rarely keep playing up until the moment you actually checkmate someone. You only play until the inevitability of that checkmate becomes clear. It might still happen 10, 15, or 30 moves down the road, but the players can see it coming.
Subscribe to Rohit Krishnan’s substack:
Real life isn’t like this. We live in a vast unpredictable Universe that we literally sculpt as we go along. There are far more variables at play than we can feasibly determine.
Once we had oracles to guide us and wise men to tell us what to do. Then the 16th century showed us the benefits of the scientific method. It enabled us to determine the strength of our paradigms by forming hypotheses and testing them through experiments. The scientific method is not a panacea or the sole arbiter of knowledge, but it is the best method we’ve found to create clarity. Well, not “get” clarity, as if it already existed in some Platonic realm, but to create it such that every day we try to be a little less wrong than the day before.
This approach only works if we try to nail down our beliefs in the form of something that can be tested or falsified. The destructiveness of nuclear weapons is something we can test, as is biological experimentation.
AI is not like that. We have a range of fears with varying degrees of realism, from the very sensible worry that we shouldn’t hook up our air traffic control to an LLM anytime soon, to the completely out-there view that an AI will become superintelligent and cause human extinction through a sort of disinterested malice.
In seeing some of these current problems and projecting them into future non-extant problems, we find ourselves in a bit of a doom loop.
What you could do is take the historic view and see this extraordinary fear, or range of fears, as the latest successor to a fine tradition of worrying about the end of the world. What we seem to be doing is taking a futuristic view and backfilling all the horrible things that might happen.
With the power of banal truisms, we’re being inevitably led to sweeping conclusions.
The concerns being raised range from the real and present to the absolutely melodramatic, which makes coherently discussing them nearly impossible. For instance, if you’re worried about the large amounts of fake news that could be generated, shouldn’t you be able to explain why we haven’t seen a deluge already? Or why will it impact the generation but not the dissemination or the consumption? Have you clicked many Taboola ads lately?
Meanwhile, taking advantage of the emerging sense of fear, the incumbents cry “Regulate us!” because regulation is the perfect moat for most providers, while both open source and a plethora of competitors wait in the wings, at most a year or two behind. Regulate us, cry those who believe the world is doomed if we pursue intelligence1, without explicitly articulating either a problem or a solution in sufficient detail.
And I keep asking, what have the models done to terrify you so? The answer is the same. Nothing, yet, but if things keep going this way we will end up in a very bad place. We conflate problems that are true now (LLMs hallucinate) with speculative problems of the far future (autonomous entities can’t be trusted). We confuse solutions today (we should work on understanding how the matrices work) with solutions for later (we should create an FDA for AI). This isn’t helpful for a meaningful conversation.
So here’s a taxonomy of the problems we talk about, and a sense of how they might be solved.
Here, I split the problems we have into three buckets: what’s true today, what might be true in the medium term, and what might be true much later. And I split what we see into four types of problems:
- Technical failures: the AI doesn’t work as intended
- Software misuse: people use the systems for bad ends
- Social externalities: the unexpected impacts from AI deployment
- Software behaves badly: autonomous AI actions cause harm
There are also two types of solutions we talk about: technological and regulatory. So, for instance, here are the problems we see from AI.
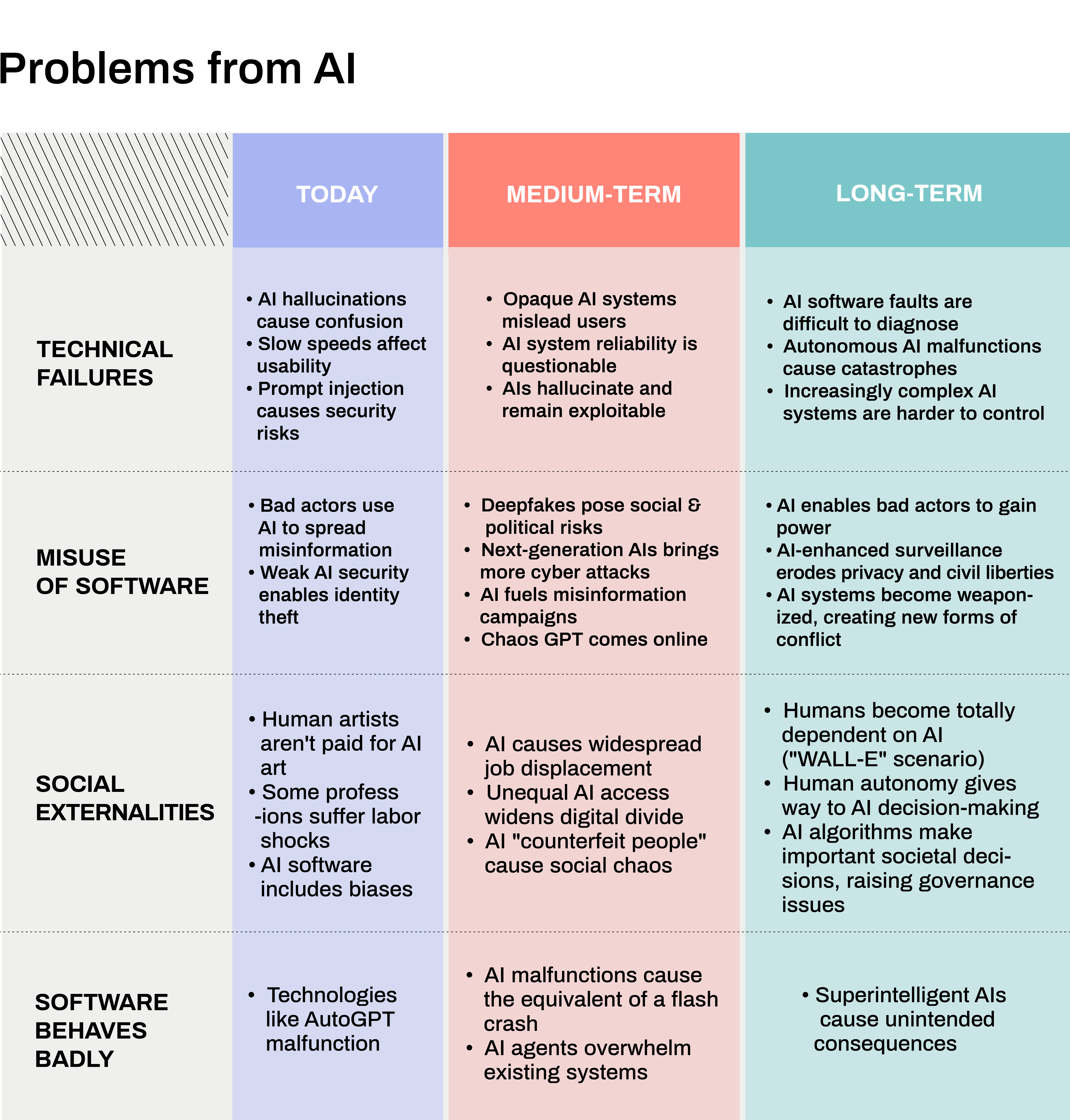
And here are the possible ways we can think about trying to solve them.
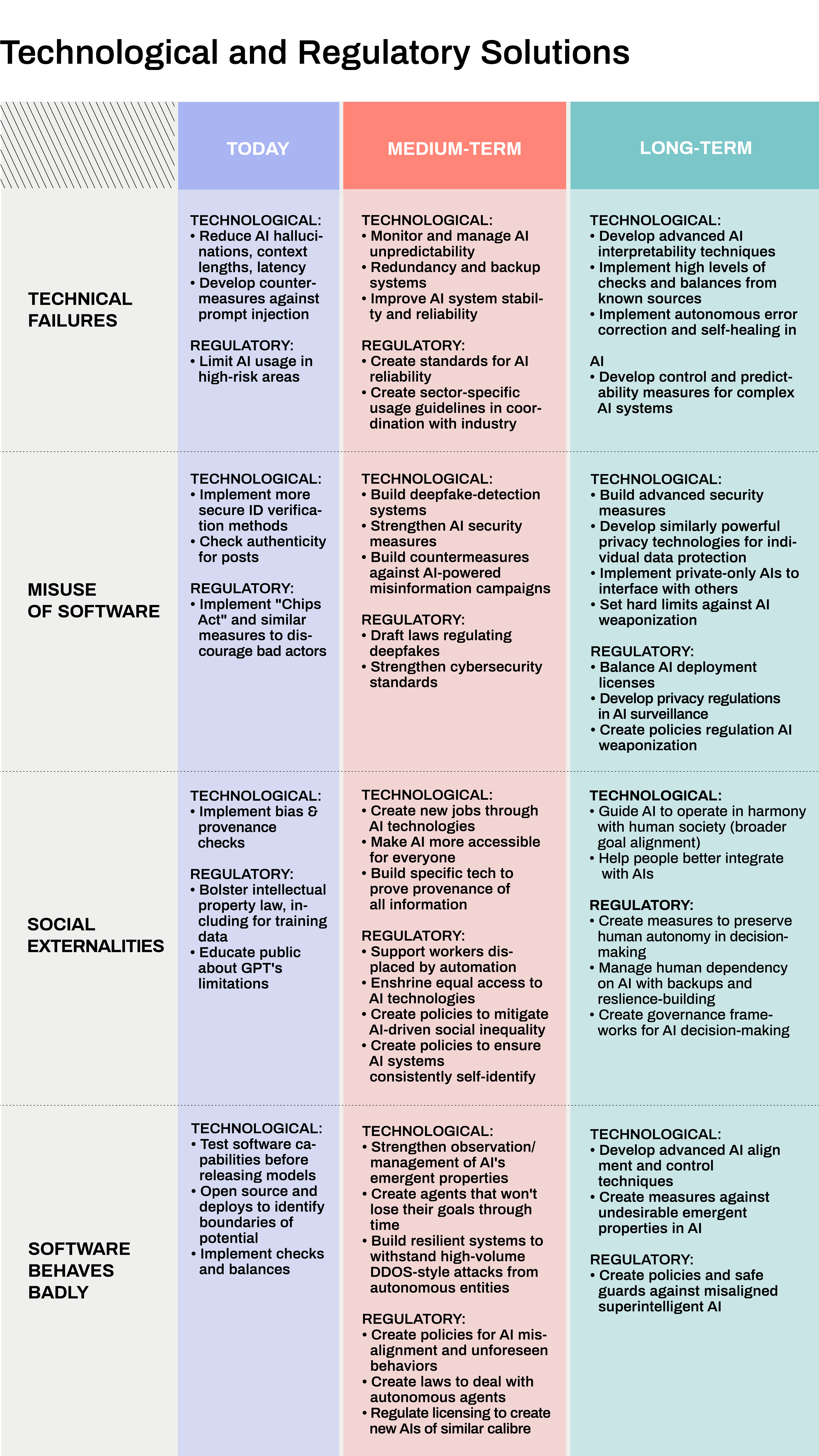
The problems are largely sensible, even if the latter ones are fanciful. And we can’t say things won’t happen, lest Popper’s ghost haunts us. But we can look at the immediate future and make plans on more concrete aspects of reality.
Exposition
A quick tour through the above matrix. Feel free to skip to the next section.
For instance, one major issue is that today’s AI systems are unreliable, and we don’t really know how exactly to make them reliable. Therefore, the answer is not to use them in places where reliability is important, right? That seems straightforward. Companies like Google and funds like RenTech have been using AI for decades and we still don’t use them even in small company decision-making. We like to find areas where getting the answer wrong isn’t immediately fatal.
If the problem is that you think companies will use them regardless of their reliability issues, may I point you to the fact that if you go to, say, a CSO (Chief Security Officer) of a large bank and tell them you have a deep-learning-enabled system that catches more malware than any other, they’ll look at you and ask, “How do you know?” If the answer isn’t empirically valid they won’t buy it. They don’t mind if the work is done by algorithms or gremlins as long as the results are solid and the price is good.
We conflate problems that are true now with speculative problems of the far future. We confuse solutions today with solutions for later.
If the problem is that you think bad actors would use it to hack systems, I’d note that identifying vulnerabilities is a multi-billion dollar industry and this would get used by white-hat hackers first, including internal security engineers. And that’s for companies, for nation-states (or large companies) they’re under a constant barrage of attack as it is2, and volume isn’t the big problem. It’s not even zero days (attacks never seen before) that cause the most damage. It’s known attacks with known vectors but nobody’s had the time to patch the systems.
Why would you think that bad actors have a unique advantage here, or that there are enough such bad actors out there to overwhelm the existing security apparatus and their ability to give their existing security engineers another tool in their arsenal? Also, if those capabilities do come to the fore, what are the chances we won’t first use them to find and patch the vulnerabilities that exist? Cybersecurity is a Red Queen’s race, where the idea isn’t perfect security but rather to stay a step ahead.
Or, what happens when the next model gets created and it is able to autonomously find vulnerabilities or give spammers power?
Same thing. The economic incentives of spam aren’t that powerful. The ROI is not very high unless the GPT calls are much cheaper, and even then it’s not particularly useful unless you can find a way to get money or secrets out of someone. Annoying people isn’t a business model3.
And if the problem you are envisioning is that we could use an agentic program, like ChaosGPT, to cause unintended mayhem, I can reassure you that they’re nowhere close to being able to do this. No matter how terrifying you find the autocomplete responses when you ask it to “please tell me how to destroy the world,” it cannot yet act coherently enough for long enough to do this. I’ve tried, as have others, and they’re frighteningly brittle.
If none of this matters, or if your concern lies with AI stealing jobs, we’re treading on familiar economic territory. We’ve been working to help those displaced by technology since we invented technology, from agriculture to manual computers! Whether it’s social safety nets or retraining, we have been looking at technology’s impact on labor markets since John Stuart Mill.
The closest societal catastrophe AI has caused is making homework essays a thing of the past.
And if the problem is that all of this is true today, but won’t be true tomorrow when we develop GPT-6, then we have to answer the extremely difficult question of what happens between today and then. Do we think we’ll learn more about how these systems work? Do we think we’ll know the boundaries of it, and when it works and when it doesn’t? Maybe not perfectly but surely we’re already getting better.
The Future
All of this brings us back to today. What we have today is an incredibly powerful and fuzzy processor. It can manipulate information in almost any format. Text, video, audio, and images can be broken down and built back up in new forms. In doing so, it hallucinates and has to be led through examples and careful phrasing. It can be taught (albeit painfully), can be given memory (though not infallibly), and can be used to great effect as our daemons.
These language models today are the reflections of our training data and methods. Their foibles are our foibles reflected back at us. And we’re getting better! GPT-4 doesn’t make the mistakes GPT-3.5 made.
We can’t know if it’s closer to a local optima where it languishes or the beginning of an exponential Moore’s law curve. We can’t know if it’s months away from sentience or decades. And we can’t know if it’s intelligent, in part because we haven’t managed to properly explain what we mean by intelligence.
We don’t even know the limits of what we’ve created well enough, and we have not seen a single instance of it actually causing any sort of widespread mayhem. Instead, the closest societal catastrophe AI has caused is making homework essays a thing of the past.
Meanwhile, it’s given us the ability to get a second opinion on anything at almost no cost, from medicine to law to taxes to food preparation, even though it’s not fully reliable yet. Today’s technology already promises a release from the great stagnation, rekindling sluggish R&D and hastening medical breakthroughs. Whether in energy, materials, or health, we advance and we improve. Blocking this path, you dam the river of progress and cast a shadow on collective well-being.
We should let ourselves use AI to make our lives better. And if it starts to seem like they are deceptive, malicious, uncaring, or prone to making plans that seem frightening to us, well we already have a plan, and plenty of nukes at the ready.
As policymakers, we have to focus on what we know. On what we can know. When we don’t know what will happen, we have to rely on models. As we saw during the recent Covid crisis, this too is liable to fail even in straightforward cases. So we tread carefully. The framework above is a way to think about what we know and separate our assumptions from what’s already happened.
Blocking this path, you dam the river of progress and cast a shadow on collective well-being.
All that said, if you’re still focused on the possible costs we might end up paying, there is one thing that I think is worth thinking about: the problem we see with widely distributed systems today. Once the use of AI systems gets widespread, we could see algorithmic flash crashes. Just like we see odd behavior and odd positive feedback loops when multiple algorithms interact in unforeseen and unforeseeable ways, like with the flash crash in the stock market, we might see similar things occur elsewhere. The mitigant I see is that AI systems are smarter than the dumb, trend-following autonomous bots that caused the flash crash, yet it remains a truism that complexity creates possible catastrophic failures.
After the flash crash happened, we started regulating high-frequency trading to stop this from happening again. We introduced circuit breakers, improved market transparency, and improved the infrastructure. This, and constant vigilance, is the price we pay to make things work better through automation. Once we knew the boundaries of how things failed, we figured out ways to work around them.
Our ancestors used to jump on ships and sail into unknown waters undeterred by the dragons that lay there. We should do no less.
Subscribe to Rohit Krishnan’s substack:
1 Which is a phrase so incredibly upsetting it makes me weep
2 The cybersecurity apparatus within companies and governments are incredibly active in thinking about this threat, and not just because of AI. They live under constant, consistent, attacks – from spearfishing emails to employees pretending to be from the CEO, to trying to sneak in malware inside, to trying to steal data.
Also, hackers normally want to steal money or secrets. Those are the places where they’ve seen success. What they haven’t done is to trick the Pentagon into thinking Russia is invading Alaska. Because a) that’s really really really hard to do, and b) we can check whether it’s true.
3 Except in some parts of the media
This article was originally published by our sister site, Freethink.