This Neural Network Is Generated Some Truly Bizarre Recipes
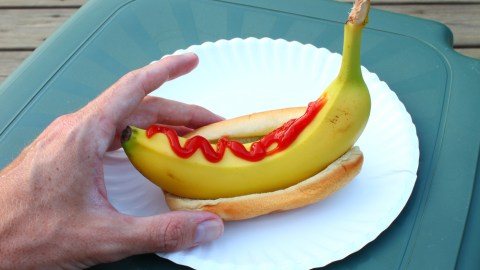
Do androids dream of cooking? That’s the question Tom Brewe asked on GitHub after he successfully trained a neural network to invent its own cookbook recipes.
Here are the ingredients for a dish called HAWAILIGELED PIE – sounds good, right?
4 Carrots; finely chopped, drained — margarine chopped 1 c Sugar, diced (optional) 1 cn Ice Cream (cleaned) Spread of fish slices 1 ea Green onions; chopped 2 oz Margarine, coarsely chopped 2 tb Sugar 1/2 c Olive oil 2 sm Eggs
The directions read “Cut into balls. The lettuce in a 10-inch baking sheet and serve with aluminum foil and dice.”
The neural network generated other recipes for dishes like BARBARA PULP0ICE, BUFTHA DINGS and CHOCOLATE RANCH BARBECUE.
Like the example above, the recipe directions are often just as absurd as the ingredients – one instructs you to beat butter until it’s smooth and then drain on both sides of the refrigerator.
How exactly does the network generate these bizarre ideas? Mostly by trial and error.
Artificial neural networks are computational models that learn by defining associations between things — images, words, letters — similar to how the human brain does. A recurrent neural network, the kind of network that was used to generate the cookbook recipes, analyzes the data it’s given (cookbook recipes, in this case) and attempts to mimic it by generating text.
It then looks at what it’s already said and uses probability to guess what to say next. The network learns through trial and error, improving with each iteration, like a toddler learning to speak.
“It’s constantly making guesses, checking its guesses, refining its own internal neuron connections based on whether it’s guessing right or now,” said Janelle Shane, a research scientist who runs the blog lewisandquarks.
Shane thought the neural network-generated recipes were hilarious, like the one that called for a half-cup of shredded bourbon. She wanted to see what other kinds of recipes a neural network could conjure.
Someone is teaching a neural network to cook. And now I can’t breathe. https://t.co/FXWrjR73b9pic.twitter.com/TqRZumTmCx
— Justin Warren (@jpwarren) March 29, 2017
So Shane found a database of cookbook recipes that and put them into char-rnn, an open-source program created by Andrej Karpathy that allows people to build their own neural networks. After inputting 30 megabytes of cookbook recipes, the network began to generate text on its own.
“My only intervention is I can set things like how many neurons does the neural network have to think with, or how are they connected to one another in a very basic sense,” Shane said.
The network's early attempts to generate recipes were little more than gibberish:
ooi eb d1ec Nahelrs egv eael
ns hi es itmyer
aceneyom aelse aatrol a
ho i nr do base
e2
o cm raipre l1o/r Sp degeedB
twis e ee s vh nean ios iwr vp e
sase
pt e
i2h8
ePst e na drea d epaesop
ee4seea .n anlp
o s1c1p , e tlsd
4upeehe
lwcc eeta p ri bgl as eumilrt
The early iterations became a bit more decipherable with time.
Immediately Cares, Heavy Mim
upe, chips
3 dill loasted substetcant
1 cubed chopped whipped cream
3 unpreased, stock; prepared; in season
1 oil
3 cup milk
1 ½ cup mOyzanel chopped
½ teaspoon lemon juice
1 ¼ teaspoon chili powder
2 tablespoon dijon stem – minced
30 dates afrester beater remaining
As the network got better at mimicking the cookbook database, Shane was curious what its first word would be.
"In this case, it’s not going to be 'mommy' or 'daddy,'" Shane said, noting that the first word the network eventually spelled correctly (after many failed attempts) was teaspoon, the most commonly used word in the input data. “It’s actually really fascinating to watch it learning a data set and see what it learns first.”
Shane's network eventually generated recipes that were coherent, and stomach-churning, with names like Completely Meat Circle, Beef Soup With Swamp Peef and Cheese and Artichoke Gelatin Dogs. Here are excerpts from some of the more bizarre recipes.
10 lb dried broccoli casserole
1 lb crab water
¼ oz can of fish casings
Place wrapper in microwave on Low Coals.
Mix honey, liquid toe water, salt and 3 tablespoon olive oil.
Cut flour into ¼-inch cubes
Spread the butter in the refrigerator.
Drop one greased pot.
Remove part of skillet.
Scrape the mold into a ball bowl.
(Illustration by Jodee Rose)
Here's one with finely grated ruck.
1 ½ teaspoon chicken brown water
1 teaspoon dry chopped leaves
1/3 cup shallows
10 oz brink custard
¼ cup bread liquid
2 cup chopped pureiped sauce
½ cup baconfroots
¼ teaspoon brown leaves
½ cup vanilla pish and sours
½ cup white pistry sweet craps
1 tablespoon mold water
¼ teaspoon paper
1 cup dried chicken grisser
15 cup dried bottom of peats
¼ teaspoon finely grated ruck
(Illustration by Jodee Rose)
Or who could resist round meat?
¼ cup white seeds
1 cup mixture
1 teaspoon juice
1 chunks
¼ lb fresh surface
¼ teaspoon brown leaves
½ cup with no noodles
1 round meat in bowl
(Illustration by Jodee Rose)
Some of Shane's favorites include a dish called Shy Sandwiches, which called for about a dozen ingredients.
"The first step is to put all the ingredients inside of a blender and process for two hours," Shane said.
There was also a recipe for a chocolate cake that actually sounded pretty reasonable, right up to the last ingredient: 1 cup of horseradish.
"A reader made that recipe and reported that it was very good, actually – unexpectedly tasty," Shane said. "So I made it and it was about the most horrible thing I've ever tried to taste. My eyes watered when I tried to open the oven."
Shane said there are plenty of researchers approaching neural networks in more sophisticated ways. IBM's Watson, for instance, has been churning out new recipes for a couple years now, combining its huge database of existing recipes with its knowledge of how chemical compounds interact to propose unexpected ingredient pairings.
"There's also a group that just recently came up with a way to change recipes from one genre to another – so, what's the French equivalent of Teriyaki chicken?" Shane said.
But the key difference between those experiments and ones conducted by hobbyists like herself?
"They aren't nearly as funny," Shane said.
She thinks the reason these recipes are funny has to do with the sheer unpredictability of the neural network. After all, it's not as if a human programmer fed the program a list of words it has to choose from to generate recipes. Rather, the network actually invents strange new words and associations as it evolves.
“That kind of freedom where it can come up with complete non-sequitur or ridiculous things, I love that sort of bizarre, almost otherworldly, creativity you get," Shane said. "It's funny.”
Shane also said there's something funny about realizing the limitations of computers. They might be magnitudes better than humans at certain things, but they fall on their faces when it comes to more nuanced tasks. Maybe there's something reassuring about knowing we have that on A.I.
(At least for now.)