Defense Department project develops first tools to detect ‘deepfake videos’
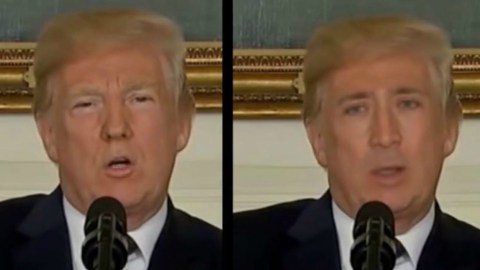
A Defense Department project has developed some of the first tools able to automatically detect a particularly deceptive type of digital content called ‘deepfake’ videos.
Deepfake videos often feature one person’s face convincingly merged with another. Other videos show one person’s face making movements and speaking words they may have never made or said in real life, so that, combined with audio manipulation, the result can be the likeness of former President Barack Obama saying things actually uttered by someone else in a studio.
The technology uses machine learning processes to learn the details of a person’s face. The A.I. analyzes video footage of the target person to learn as much as it can; the more footage it has to study, the more it learns. That’s why presidents and celebrities are frequently used in deepfake experiments.
It’s a technological evolution that’s alarmed many in media and government, unsurprisingly. The fear is that it could usher in a new era of fake news, one in which it would be virtually impossible to tell whether what you see on a screen is real or fake.
“This is an effort to try to get ahead of something,” said Florida senator Marco Rubio in remarks at the Heritage Foundation. “The capability to do all of this is real. It exists now. The willingness exists now. All that is missing is the execution. And we are not ready for it, not as a people, not as a political branch, not as a media, not as a country.”
In 2014, deepfake technology started to get much better thanks to an innovative approach called generative adversarial networks (GAN). As Martin Giles writes for the MIT Technology Review, the approach is similar to an art forger and an art detective who repeatedly try to outwit one another, resulting in increasingly convincing fakes.
“Both networks are trained on the same data set. The first one, known as the generator, is charged with producing artificial outputs, such as photos or handwriting, that are as realistic as possible. The second, known as the discriminator, compares these with genuine images from the original data set and tries to determine which are real and which are fake. On the basis of those results, the generator adjusts its parameters for creating new images. And so it goes, until the discriminator can no longer tell what’s genuine and what’s bogus.”
Recently, a contest run by the U.S. Defense Advanced Research Projects Agency (DARPA) asked researchers to automate existing forensic tools in an effort to keep up with deepfake technology. The goal was to find areas where deepfake technology is falling short.
One forensics approach exploited a rather simple observation: faces created by deepfake technology hardly ever blink. The reason? Neural networks that create the deepfakes typically study still images of a target face, not blinking ones. It’s a good exploit for now, but deepfake generators could get around it by feeding the networks more images of blinking faces.
Other techniques, as Will Knight wrote for MIT Technology Review, take advantage of the peculiar signatures deepfake technology leaves behind, like unnatural head movements and odd eye color.
“We are working on exploiting these types of physiological signals that, for now at least, are difficult for deepfakes to mimic,” says Hany Farid, a leading digital forensics expert at Dartmouth University.
Still, it’s possible these kinds of forensic approaches will be forever one step behind the evolution of deepfake technology.
“Theoretically, if you gave a GAN all the techniques we know to detect it, it could pass all of those techniques,” David Gunning, the DARPA program manager in charge of the project, told MIT Technology Review. “We don’t know if there’s a limit. It’s unclear.”