Predictive policing: Data can be used to prevent crime, but is that data racially tinged?
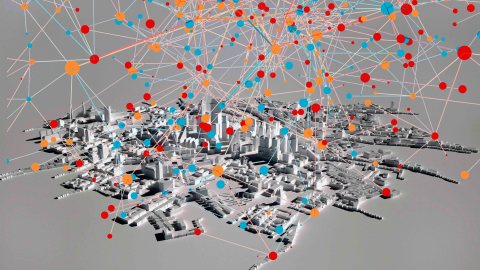
As predictive analytics advances decision making across the public and private sectors, nowhere could this prove more important – nor more risky – than in law enforcement. If the rule of law is the cornerstone of society, getting it right is literally foundational. But the art of policing by data, without perpetuating or even magnifying the human biases captured within the data, turns out to be a very tricky art indeed.
Predictive policing introduces a scientific element to law enforcement decisions, such as whether to investigate or detain, how long to sentence, and whether to parole. In making such decisions, judges and officers take into consideration the calculated probability a suspect or defendant will be convicted for a crime in the future. Calculating predictive probabilities from data is the job of predictive modeling (aka machine learning) software. It automatically establishes patterns by combing historical conviction records, and in turn these patterns – together a predictive model – serve to calculate the probability for an individual whose future is as-yet unknown. Such predictive models base their calculations on the defendant’s demographic and behavioral factors. These factors may include prior convictions, income level, employment status, family background, neighborhood, education level, and the behavior of family and friends.
Ironically, the advent of predictive policing came about in part to address the very same social justice infringements for which it’s criticized. With stop and frisk and other procedures reported to be discriminatory and often ineffective, there emerged a movement to turn to data as a potentially objective, unbiased means to optimize police work. Averting prejudice was part of the impetus. But the devil’s in the detail. In the process of deploying predictive policing and analyzing its use, complications involving racial bias and due process revealed themselves.
The first-ever comprehensive overview, The Rise of Big Data Policing: Surveillance, Race, and the Future of Law Enforcement, strikes an adept balance in covering both the promise and the peril of predictive policing. No one knows how much of a high wire act it is to justly deploy this technology better than the book’s author, law professor Andrew Guthrie Ferguson. The book’s mission is to highlight the risks and set a cautionary tone – however, Ferguson avoids the common misstep of writing off predictive policing as an endeavor that will always intrinsically stand in opposition to racial justice. The book duly covers the technical capabilities, underlying technology, historical developments, and numerical evidence that support both its deployed value and its further potential (on a closely-related topic, I covered the analogous value of applying predictive analytics for homeland security).
The book then balances this out by turning to the pitfalls, inadvertent yet dire threats to civil liberties and racial justice. Here are some of the main topics the book covers in that arena.
Racial Bias
As Ferguson puts it, “The question arises about how to disentangle legacy police practices that have resulted in disproportionate numbers of African American men being arrested or involved in the criminal justice system… if input data is infected with racial bias, how can the resulting algorithmic output be trusted?” It turns out that predictive models consulted for sentencing decisions falsely flag black defendants more often than white defendants. That is, among those who will not re-offend, the predictive system inaccurately labels black defendants as higher-risk more often than it does for white defendants. In what is the most widely cited piece on bias in predictive policing, ProPublica reports that the nationally used COMPAS model (Correctional Offender Management Profiling for Alternative Sanctions) falsely flags black defendants at almost twice the rate of white defendants (44.9% and 23.5%, respectively). However, this is only part of a mathematical conundrum that, to some, blurs the meaning of “fairness.” Despite the inequity in false flags, each individual flag is itself racially equitable: Among those flagged as higher risk, the portion falsely flagged is similar for both black and white defendants. Ferguson’s book doesn’t explore this hairy conundrum in detail, but you can learn more in an article I published about it.
Ground Truth: One Source of Data Bias
The data analyzed to develop crime-predicting models includes proportionately more prosecutions of black criminals than white ones and, conversely, proportionately fewer cases of black criminals getting away with crime (false negatives) than of white criminals. Starting with a quote from the ACLU’s Ezekiel Edwards, Ferguson spells out why this is so:
“Time and again, analysis of stops, frisks, searches, arrests, pretrial detentions, convictions, and sentencing reveal differential treatment of people of color.” If predictive policing results in more targeted police presence, the system runs the risk of creating its own self-fulfilling prediction. Predict a hot spot. Send police to arrest people at the hot spot. Input the data memorializing that the area is hot. Use that data for your next prediction. Repeat.
Since the prevalence of this is, by definition, not observed and not in the data, measures of model performance do not reveal the extent to which black defendants are unjustly flagged more often. After all, the model doesn’t predict crime per se; it predicts convictions – you don’t know what you don’t know. Although Ferguson doesn’t refer to this as a lack of ground truth, that is the widely used term for this issue, one that is frequently covered, e.g., by The Washington Post and by data scientists.
Constitutional Issues: Generalized Suspicion
A particularly thorny dispute about fairness – that’s actually an open constitutional question – arises when predictive flags bring about searches and seizures. The Fourth Amendment dictates that any search or seizure be “reasonable,” but this requirement is vulnerable to corruption when predictive flags lead to generalized suspicion, i.e., suspicion based on bias (such as the individual’s race) or factors that are not specific to the individual (such as the location in which the individual finds him- or herself). For example, Ferguson tells of a black driver in a location flagged for additional patrolling due to a higher calculated probability of crime. The flag has placed nearby a patrol, who pulls over the driver in part due to subjective “gut” suspicion, seeing also that there is a minor vehicle violation that may serve to explain the stop’s “reasonableness”: the vehicle’s windows are more heavily tinted than permitted by law. It’s this scenario’s ambiguity that illustrates the dilemma. Do such predictive flags lead to false stops that are rationalized retroactively rather than meeting an established standard of reasonableness? “The shift to generalized suspicion also encourages stereotyping and guilt by association. This, in turn, weakens Fourth Amendment protections by distorting the individualized suspicion standard on the street,” Ferguson adds. This could also magnify the cycle perpetuating racial bias, further corrupting ground truth in the data.
Transparency: Opening Up Otherwise-Secret Models that Help Determine Incarceration
Crime-predicting models must be nakedly visible, not amorphous black boxes. To keep their creators, proponents, and users accountable, predictive models must be open and transparent so they’re inspectable for bias. A model’s inner workings matter when assessing its design, intent, and behavior. For example, race may hold some influence on a model’s output by way of proxies. Although such models almost never input race directly, they may incorporate unchosen, involuntary factors that approximate race, such as family background, neighborhood, education level, and the behavior of family and friends. For example, FICO credit scores have been criticized for incorporating factors such as the “number of bank accounts kept, [which] could interact with culture – and hence race – in unfair ways.”
Despite this, model transparency is not yet standard. For example, the popular COMPAS model, which informs sentencing and parole decisions, is sealed tight. The ways in which it incorporates such factors is unknown – to law enforcement, the defendant, and the public. In fact, the model’s creators recently revealed it only incorporates a selection of six of the 137 factors collected, but which six remains a proprietary secret. However, the founder of the company behind the model has stated that, if factors correlated with race, such as poverty and joblessness, “…are omitted from your risk assessment, accuracy goes down” (so we are left to infer the model may incorporate such factors).
In his book, Ferguson calls for accountability, but stops short of demanding transparency, largely giving the vendors of predictive models a pass, in part to protect “private companies whose business models depend on keeping proprietary technology secret.” I view this allowance as inherently contradictory, since a lack of transparency necessarily compromises accountability. Ferguson also argues that most lay-consumers of model output, such as patrolling police officers, would not be equipped to comprehend a model’s inner workings anyway. However, that presents no counterargument to the benefit of transparency for third party analytics experts who may serve to audit a predictive model. Previously, before his book, Ferguson had influenced my thinking in the opposite direction with a quote he gave me for my writing (a couple years before his book came out). He told me, “Predictive analytics is clearly the future of law enforcement. The problem is that the forecast for transparency and accountability is less than clear.”
I disagree with Ferguson’s position that model transparency may in some cases be optional (a position he also covers in an otherwise-valuable presentation accessible online). This opacity infringes on liberty. Keeping the inner workings of crime-predictive models proprietary is like having an expert witness without allowing the defense to cross-examine. It’s like enforcing a public policy the details of which are confidential. There’s a movement to make such algorithms transparent in the name of accountability and due process, in part forwarded by pertinent legislation in Wisconsin and in New York City, although the U.S. Supreme Court declined to take on a pertinent case last year.
Deployment: It’s How You Use It that Matters
In conclusion, Ferguson lands on the most pertinent point: It’s how you use it. “This book ends with a prediction: Big data technologies will improve the risk-identification capacities of police but will not offer clarity about appropriate remedies.” By “remedy,” this lawyer is referring to the way police respond, the actions taken. When it comes to fairness in predictive policing, it is less the underlying number crunching and more the manner in which it’s acted upon that makes the difference.
Should judges use big data tools for sentencing decisions? The designer of the popular COMPAS crime-predicting model did not originally intend it be used this way. However, he “gradually softened on whether this could be used in the courts or not.” But the Wisconsin Supreme Court set limits on the use of proprietary scores in future sentencing decisions. Risk scores “may not be considered as the determinative factor in deciding whether the offender can be supervised safely and effectively in the community.”
To address the question of how model predictions should be acted upon, I urge law enforcement to educate and guide decision makers on how big data tools inevitably encode racial inequity. Train judges, parole boards, and officers to understand the pertinent caveats when they’re given the calculated probability a suspect, defendant, or convict will offend or reoffend. In so doing, empower these decision makers to incorporate such considerations in whatever manner they deem fit – just as they already do with the predictive probabilities in the first place. See my recent article for more on the considerations upon which officers of the law should reflect.
Ferguson’s legal expertise serves well as he addresses the dilemma of translating predictions based on data into police remedies – and it serves well throughout the other varied topics of this multi-faceted, well-researched book. The Amazon description calls the book “a must read for anyone concerned with how technology will revolutionize law enforcement and its potential threat to the security, privacy, and constitutional rights of citizens.” I couldn’t have put it better myself.
—
Eric Siegel, Ph.D., founder of the Predictive Analytics World and Deep Learning World conference series – which include the annual PAW Government – and executive editor of The Predictive Analytics Times, makes the how and why of predictive analytics (aka machine learning) understandable and captivating. He is the author of the award-winning Predictive Analytics: The Power to Predict Who Will Click, Buy, Lie, or Die, a former Columbia University professor, and a renowned speaker, educator, and leader in the field.