Why We Can Predict A Lot About the Next Mass Shooting—But Never Enough to Prevent It
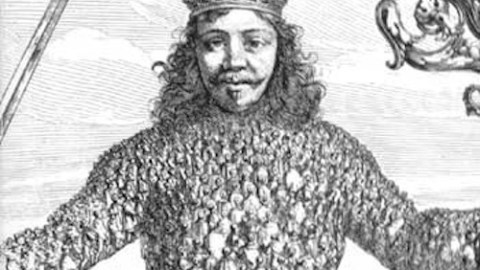
“I do not know whether I shall return from my long weekend trip alive,” the mathematical psychologist Anatol Rapoport once wrote. “But I do know that the number of traffic victims will be between 400 and 700.” Similarly, I do not know if some schlubby white guy in his 30s will gun down a bunch of people at work in Spokane next February 12, as Philip Bump writes over at Atlantic Wire. But Bump can confidently predict that there will be a mass shooting next year with all those characteristics. That’s his real point. As would any sane person, he acknowledges the unbridgeable chasm between patterns in data and the uniqueness of a single event, of a single person—that unpredictability of specific events which makes it almost certain there will not be a Spokane Massacre on February 12. Seeing this chasm is easy if you focus hard, but it doesn’t come naturally. That’s the major downside to the ever-growing use of Big Data to understand ourselves and our problems.
There’s no contradiction between the fact that mass behavior is predictable even as no one person’s actions are. Rapoport explains it with the analogy of a cloud full of water vapor. The motion of each water molecule is random with respect to other water molecules. But if the cloud is being blown along by a strong wind, you can predict where all those water molecules, together, will be in an hour. Individual unpredictability nestles within collective patterns.
Now imagine that each water molecule is bouncing around thinking thoughts like “should I buy more Apple shares?” or “let’s have another kid!” or “burger or salad?” How do you get them to believe that the wind will take them where they are going, regardless of their little choices (a psychological issue)? More importantly, how do you answer a molecule that says, “whatever happens to this cloud, you can’t predict where I’ll end up!” (which is, in fact, true) ?
There aren’t any good answers that I know, which is why politics seems so often to choke on statistical inferences. Give people a data analysis, they’ll demand that a statistical probability about many people be treated as a dead certainty about one person (“he fits the profile, he must be a terrorist”). Or they go the opposite way, invoking the independence of individuals from general patterns (“I smoked for 10 years, never sick a day in my life”).
Consider climate change. After every Katrina or Sandy, activists are eager to tell the public that global warming caused the catastrophe. It drives them crazy when climate scientists point out that you can’t draw a straight causal line from climate change to any single particular event. Yes, climate change makes extreme weather more likely. But climate change cannot be said to have flooded your house in Brooklyn. People are invariably more impressed by “I pushed it and it moved” type causes than they are by “odds are, this will happen some day.” It would be great, then, to bridge the gap between probabilities involving events and a particular hurricane or flood. Too bad it can’t be done.
Or consider people’s feelings about privacy and public safety. Intelligence officials tell us that in order to find a needle in a haystack, they need a haystack—vast amounts of data about people’s Internet surfing, emails, and phone calls. In the abstract, it sounds harmless—a big pile of numbers from which computers can extract patterns. But when you imagine it as an individual, the thought of a computer tracking you is an intolerable intrusion. Similarly, New York’s data-happy mayor can defend its “stop-and-frisk” policy with talk of “math” and “logic.” But the tactic still became politically radioactive because of its effect on individuals who both (a) fit a pattern in crime data and (b) had not done anything wrong.
Or take another data-driven initiative of Mayor Bloomberg—his anti-obesity measures. Will any single giant soda give a citizen diabetes? Will any single pack of cigarettes give you lung cancer? Nope and nope. Can statistics even say that Joe Blow of 123 East 345th Street had a heart attack because of fatty foods or cigarettes? They cannot. Statistics can say that Mr. Blow had a higher risk of death because of his habits. But “greater likelihood” is not the kind of “push-it-and-it-moves” causation that convinces people and makes them change.
Education isn’t enough to overcome such strong biases. After all, the germ theory of disease has been around the more than 100 years, and has greatly changed people’s behavior, even though it runs counter to our innate intuitions (human beings instinctively avoid bad smells, creepy-crawling bugs and scummy liquids, but they have to be trained to avoid passing bacteria and viruses that they can’t sense). Despite all that history and knowledge, though, doctors stilldon’t wash their hands enough. Years of training isn’t a perfect bulwark against the feeling of “it won’t happen to me.”
Germs are tiny invisible causes of illness that our minds still haven’t totally mastered. Big Data is increasingly going to reveal gigantic invisible causes of behavior, and I think we won’t master those facts any more easily. Data will tell us significant things about human behavior in general, and about the effects of global warming, fracking, overfishing and other large scale activities. And as long as its analysis applies to strangers, we’ll be all for the quantitative approach. But when the data analysis touches our own lives, our own practices, we will say “your statistics don’t describe me!”
At the end of War and Peace, Tolstoy asserts that people just aren’t capable of accepting that each person’s uniqueness doesn’t matter:
Just as in astronomy the difficulty of admitting the motion of the earth lay in the immediate sensation of the earth’s stationariness and of the planets’ motion, so in history the difficulty of recognizing the subjection of the personality to the laws of space and time and causation lies in the difficulty of surmounting the direct sensation of the independence of one’s personality.
That may be a psychological defense mechanism, but it also happens to be the literal truth. Given the number of 21st-century problems that are visible only in data, the chasm between Aggregate and Individual is going to be a problem for a long time to come.
Follow me on Twitter: @davidberreby