How AI Learned to Bluff and Beat Humans at Poker
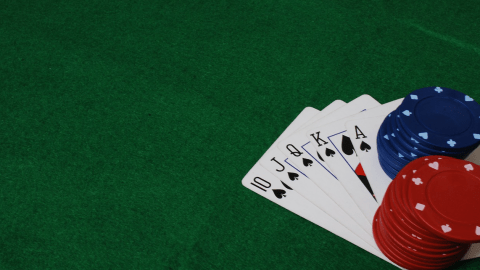
How about a nice game of chess?
The list of recent defeats where humans were overmatched by machines are well-known: chess champion Garry Kasparov losing against IBM’s Deep Blue, Jeopardy wiz Ken Jennings being soundly defeated by IBM’s Watson, and Go champion Lee Sodol losing to Google’s AlphaGo.
We may also be able to add poker to the list of AI superiority.
Professional poker player Jason Les playing against Libratus, an AI program.
A recent twenty-day competition between poker champions (heads-up no-limit Texas hold’em, 120,000 total hands) and Libratus, an AI program created by Carnegie Mellow University professors Tuomas Sandholm and Noam Brown, had the AI coming out on top. This is particularly surprising because unlike games like chess and Go, where the information is upfront and know (“Perfect Information Games”), poker involves a great deal of hidden information (“Imperfect Information Games”) and the seemingly-human characteristic of bluffing. It turns out that AI can learn the art of bluffing.
This year, Libratus became the first AI to defeat poker champions in heads-up no-limit Texas hold’em poker.
“It wasn’t just a matter of figuring out a strategy versus a static opponent, it ended up changing its strategy as time went on.”-Jason Les, professional poker player
Why is Poker So Difficult for AI to Master?
AI benefits from figuring out a strategy based on rules and known information, and poker included a great deal of hidden information. Unlike a chessboard displaying your opponent’s chess pieces, your opponent’s hand in poker is hidden. Poker has a near-infinite amount of possible situations–10 to the 160th power to be exact. That’s greater than the number of atoms in the universe.
Libratus has a great deal of computer power running it, connected to the Pittsburgh Supercomputer Center. Instead of being taught the best way to play poker–which would be relevant for a Perfect Information Game like chess, checkers, or Go–Libratus was taught the rules of poker and then learned through its interactions with the human players. The AI was given a reward function to win as much money as possible and then instructed to optimize the reward function. (The co-creator of Libratus, Professor Noam Brown of Carnegie Mellon, explains how the AI was programmed in a Software Engineering Daily podcast).
Libratus was constructed by first solving an abstraction of the game via a new variant of Monte Carlo CFR that samples negative-regret actions less frequently. Libratus applied nested subgame solving upon reaching the third betting round, and in response to every subsequent opponent bet thereafter. This allowed Libratus to avoid information abstraction during play, and leverage nested subgame solving’s far lower exploitability in response to opponent off-tree actions.-Safe and Nested Subgame Solving for Imperfect-Information Games, Noam Brown and Tuomas Sandholm
In other words, Libratus learned the subtle flaws in the poker champions’ play and began capitalizing on it. While the humans-versus-Libratus event was billed as Brains Versus Artificial Intelligence, it may be better to think it as Human Brains versus AI Brains.
AI Can Beat Poker Champions. So What?
Unlike mastering a set of rules–what IBM’s Deep Blue did for chess and Google’s AlphaGo did for Go–the success of Libratus may indicate a potential future where AI assists humans in tasks involving negotiation and other situations where the available facts are incomplete.
“It is a really critical milestone in developing AIs that can solve real world problems with incomplete information, which are the ones we need to solve to advance society–not just poker.”-Nick Nystrom, Senior Director of Research at the Pittsburgh Supercomputer Center (speaking to Engadget)
Similar to how IBM’s Watson went from an expensive parlor trick on Jeopardy to assisting business decisions, today’s poker champion can be tomorrow’s business engine.