To Win with Algorithms, We Need to Keep Score of Humans
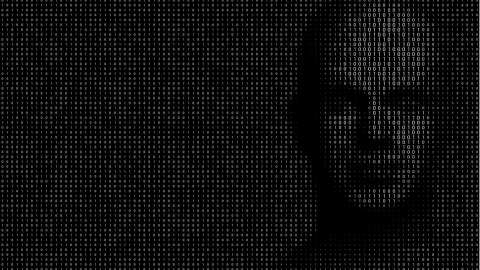
More and more companies are incorporating machine-based intelligence in decision-making processes. Computers are great at numbers and finding meaningful trends in big data, so this makes sense. On the other hand, they’re not always as good at recognizing big-picture things that can be obvious to humans. So it makes sense to retain the option of overriding algorithm-based decisions. As Andrew McAfee of MIT Center for Digital Business says, “I’m a big fan of automated medical diagnoses…But I don’t think that if I got diagnosed with cancer tomorrow, I would want a digital oncologist that no human being could intervene on top of or could override.” Human judgment continues to have a role in automated decision-making. But how can we know if we should intervene?
In his video “Building Mind-Machine Combinations: Keep Score to Improve Decision Quality” for Big Think+, McAfee proposes what he claims is a critical prerequisite for effectively collaborating with our intelligent machines: We need to first start consistently tracking our own decision-making record.
Keeping score
We humans make a lot of decisions based on our intuition and our gut — they can seem to cut to the truth in a way our conscious decision-making processes can’t. McAffee respects such human gifts, but says we still need to objectively measure the degree to which our ways of thinking help us find solutions to problems, or not. Concerned that we often award trust to individuals more readily than we really ought to, he asks, “Are we just remembering that four product cycles ago that person had a great idea, and that’s what sticks in our minds, so we continue to listen to them?”
More sensible, McAfee says, is to track each individuals’ past decisions and predictions to see how they actually turned out. His intent isn’t to judge an employee’s worth — we simply want to know how successful his or her decisions have been. In addition to identifying our best decision-makers, assessing a person’s success rate offers the added benefit of helping them improve. As McAfee says, “If we “show them the examples they got wrong…maybe they can lower their error rate.”
More productive human/machine collaboration
Even more critically, scoring can help us better leverage machine intelligence by objectively assessing the real value of intervention in its decisions. When humans reject automated results, McAfee says, it’s vital that we keep score and “watch over time if when human beings override what the algorithm says, do they override with a lower error rate or a higher rate?” After all, the value of human intervention is to increase the quality of decisions. “It’s a pretty straightforward thing to do,” he points out, “and over time we’ll learn if those interventions are effective or not, or if that person is intervening appropriately or not.”
More than relying on “experts,” more than relying on reputations, McAfee’s video makes a compelling case that keeping score is a key element in realizing our dream of effective, rewarding human/machine collaboration.