Is this AI research preliminary? How to think critically about eye-catching studies
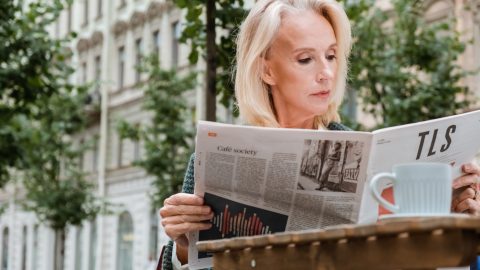
Smiling makes us feel happier. If you strike a superhero pose, you’ll feel more assertive and confident in your abilities. We all have a limited pool of self-control that can be exhausted through use. Imperceptible subconscious cues have outsized effects on people’s later behaviors.
You may be familiar with these psychological phenomena—known as the facial-feedback hypothesis, power posing, ego depletion, and social priming respectively. Thanks to TED talks, bestselling books, and pop-science websites, these ideas have escaped the ivy-covered halls to become part of our social lexicon and collective consciousness. They may also be bull.
Researchers’ attempts to replicate these, and other psychological hypotheses, have come up short. This event, known as the “Replication Crisis,” has led many in psychology to question their study designs and potential publication bias.
While the current crisis lies in psychology, the field does not stand alone. Economics and medical research have endured their own bouts with replication. And one field ripe for an upcoming replication crisis is artificial intelligence research.
In this video preview, psychologist Gary Marcus, author of Rebooting AI, explains a critical component of scientific research and shares essential questions for evaluating studies.
One Study Does Not Rule Them All
- Replicability Crisis: An ongoing methodological crisis in which many scientific studies are found difficult or impossible to reproduce.
- Sometimes a single study that’s interesting gets reported as fact. But that doesn’t mean it really is. Statistics suggest that about 50% of the studies in major publications don’t replicate.
- To get to the truth of a research question, multiple studies are needed. A meta-analysis combines multiple studies to look for overall trends.
Unless your keynote-speaking career is on the line, the Replication Crisis isn’t much of a crisis. Not really.
Marcus points out that truth is not determined by a single study. Instead, researchers repeat experiments to see if earlier results can be falsified. They devise new experiments to test old hypotheses, and they develop new hypotheses that may explain observations better. These new hypotheses, of course, must be subjected to study and replication as well.
In this way, scientists collect many results over time. Only when these results have been combined and statistically analyzed—through a process called meta-analysis—can we begin to say whether a hypothesis is credible. Even then, any hypothesis remains open to questioning, testing, and adjusting based on new data.
This process is why the Replication Crisis doesn’t suggest psychology is an untrustworthy field. Quite the opposite: It’s science operating as intended.
It’s also worth noting that many other psychological phenomena have held up to refutation through replication. These sturdier (for now) ideas include that personality traits remain stable throughout our lives, group beliefs shape personal beliefs, and people overestimate predictability after the fact.
A Critical Eye Toward AI
- Did the researchers reveal the conditions of their experiment?
- Were they able to produce the results more than once?
- Did the researchers report all of the results, or just the most exciting ones?
- Did the researchers define in advance what they were going to be testing statistically?
If we’re going to bring science into our organizations, then we need to learn to evaluate studies and their results using a scientific mindset.
Enter artificial intelligence. AI is on track to reshape our world in major ways. That forecast means most, if not all, businesses will need to tackle the question of AI—if not now then soon. It also means a lot of enthusiasm for results and the desire to push the current limits.
Unfortunately, this digital gold rush can lead to scientific shortcuts on the research side. This is especially true in the way study results are reported in journals, at conferences, in marketing materials, and, of course, news outlets. Researchers are incentivized for career purposes to promote sexy results. Journals will favor confirmatory results that may skew long-term understanding. And reporters may write on a topic they don’t fully comprehend but think is super cool.
For all these reasons, we need to create a deeper understanding of scientific research. We can’t scan headlines on our newsfeed and pretend we know what’s happening. We need to read the original studies, scrutinize their data and findings, and be willing to compare the results to others in the field. Whether its AI, psychology, or some other field, Marcus’s questions are a good place to start.
Ready or not, AI is here and the changes are just beginning. With video lessons ‘For Business‘ from Big Think+, you can better prepare your team for the new paradigm. Gary Marcus joins more than 350 experts to teach lessons in data analysis and disruptive technology:
- Is This Research Preliminary?: Why We Need to Look Critically at Eye-Catching Studies
- Can We Generalize to the Population?: Why We Should Scrutinize Sampling Methods
- What Is the Nature of This Relationship?: Why Correlation Does Not Imply Causation
- Proceed with Caution: Help Your Organization Help AI Change the World
Request a demo today!